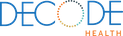
Decode Health
Founded Year
2019Stage
Spinoff / Spinout | AliveMosaic Score The Mosaic Score is an algorithm that measures the overall financial health and market potential of private companies.
-212 points in the past 30 days
About Decode Health
Decode Health specializes in providing an AI platform as a service (PaaS) that focuses on precision medicine within the healthcare sector. The company offers solutions for pharmaceutical, diagnostic, and technology companies by leveraging multiomics, clinical data, social determinants of health, and other healthcare data sources to deliver actionable insights and accelerate research and development. Decode Health primarily serves the pharmaceutical industry, diagnostic research, and technology platforms seeking to enhance disease management and outcomes. It was founded in 2019 and is based in Nashville, Tennessee.
Loading...
Decode Health's Product Videos
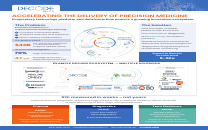
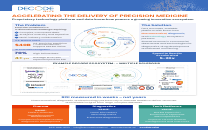
Decode Health's Products & Differentiators
Decode Health Analytics Platform
Dynamic design framework powered by technologies that cover many data, target, and methodology inputs routed through robust machine-learning modeling to produce the best predictions.
Loading...
Decode Health Patents
Decode Health has filed 1 patent.
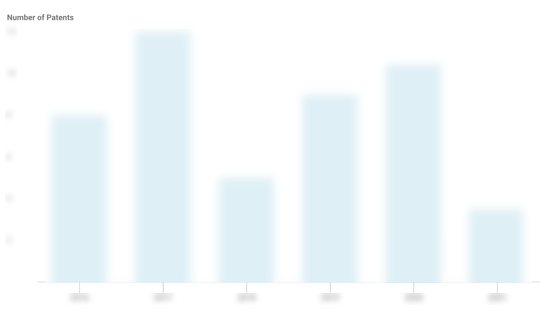
Application Date | Grant Date | Title | Related Topics | Status |
---|---|---|---|---|
6/18/2018 | 7/25/2023 | RNA, Autoimmune diseases, Transcription factors, MicroRNA, Molecular biology | Grant |
Application Date | 6/18/2018 |
---|---|
Grant Date | 7/25/2023 |
Title | |
Related Topics | RNA, Autoimmune diseases, Transcription factors, MicroRNA, Molecular biology |
Status | Grant |
Latest Decode Health News
Sep 16, 2024
Abstract Shortening of telomere length (TL) is correlated with many age-related disorders and is a hallmark of biological aging. This study used proteome-wide Mendelian randomization to identify the protein biomarkers associated with telomere length. Protein quantitative trait loci (pQTL) were derived from two studies, the deCODE Health study (4907 plasma proteins) and the UK Biobank Pharma Proteomics Project (2923 plasma proteins). Summary data from genome-wide association studies (GWAS) for TL were obtained from the UK Biobank (472,174 cases) and GWAS Catalog (418,401 cases). The association between proteins and TL was further assessed using colocalization and summary data-based Mendelian randomization (SMR) analyses. The protein–protein network, druggability assessment, and phenome-wide MR were used to further evaluate the potential biological effects, druggability, and safety of the target proteins. Proteome-wide MR analysis identified 22 plasma proteins that were causally associated with telomere length. Five of these proteins (APOE, SPRED2, MAX, RALY, and PSMB1) had the highest evidence of association with TL and should be prioritized. This study revealed telomere length-related protein biomarkers, providing new insights into the development of new treatment targets for chronic diseases and anti-aging intervention strategies. Introduction Telomeres are located at the termini of every linear chromosome and are a specific type of DNA–protein complex. Telomeres and their associated proteins protect chromosomal ends to maintain genomic stability. During DNA replication, the ends of chromosomes cannot be completely replicated 1 , leading to the progressive shortening of telomeres with cell division and ultimately to cellular senescence 2 . Therefore, telomere length (TL) shortening has long been defined as a sign of senescence 3 , 4 . Several studies have demonstrated an association between shortened telomere length (often assessed using leukocyte TL) and human longevity and mortality 5 , 6 . Shortened telomeres have also been associated with an increased likelihood of age-associated diseases, including cardiovascular disease 7 , type 2 diabetes 8 , and some neurological disorders 9 . Many factors affect TL, including lifestyle, nutrition, environmental exposure 10 , and genetics. For example, the relationship between TL and lifestyle factors (e.g., smoking and obesity) has been investigated 11 , 12 . TL is also affected by genetic variants [e.g., single nucleotide polymorphisms (SNPs)] 13 . It has also been shown that oxidative DNA damage can lead to telomere wear and tear or dysfunction, which can trigger cellular senescence and apoptosis 14 . Previous research has indicated that TL is also regulated by proteins such as WRAP53, which affects TL by regulating telomerase activity 15 , and other proteins that participate in cell cycle-related signaling pathways 16 , 17 . However, considering the limitation of the research scope, a large percentage of TL-related proteins have not yet been fully explored. Therefore, further insight into the molecular mechanisms of telomere maintenance is important for improving human health and developing therapeutic strategies against a number of age-related diseases. Mendelian randomization (MR) analyses use genetic instrumental variables (IVs), specifically SNPs derived from genome-wide association studies (GWAS), to assess the causal impact of exposure on outcomes through genetic variation 18 . Based on the plasma protein data derived from two studies 19 , 20 , we first conducted a systematic study on the causal relationship between thousands of plasma proteins and TL using MR analysis. Sensitivity analyses and Steiger directionality tests were performed to exclude the effects of confounding factors and reverse causality. Subsequently, the level of association between the proteins and the TL was prioritized by integrating the results of repeatability MR, Bayesian colocalization, and summary data-based MR (SMR) analysis. In addition, the biological functions and potential interactions between proteins were identified through Gene Ontology enrichment analysis and protein–protein interaction (PPI) networks. Furthermore, we assessed the potential pleiotropy and side effects of the identified target proteins using a druggability evaluation and phenome-wide MR. This study aimed to identify protein markers related to TL and provide new information for future research on potential therapeutic targets for chronic diseases to improve healthy aging. Methods The overall framework of the study design is shown in Fig. 1 . This study used summary data derived from two proteomics studies to investigate the correlation between plasma proteins and TL using two-sample MR. Fig. 1 Exposure data sources We selected cis-protein quantitative loci (pQTL) from two GWAS datasets, the deCODE Health Study 20 and the UK Biobank Pharma Proteomics Project (UKB-PPP) 19 , as genetic instrumental variables for plasma proteins. The UKB-PPP collected data on 2923 proteins via proteomic analysis of 54,219 plasma samples through the Olink platform. From these, we extracted cis-pQTL from 1314 plasma proteins as IVs for MR analysis. The deCODE Health study measured 4907 proteins in the plasma of 35,559 Icelanders using the SomaScan platform and found 18,084 correlated sequence variants between plasma protein levels. We extracted cis-SNPs from 1297 plasma proteins as IVs for the MR analysis. For plasma proteins that overlapped in the two studies, the protein exhibiting the highest total R2 was selected for retention. Data sources of telomere length data The main outcome data for this study were derived from the GWAS dataset, which is accessible to the public 21 . This dataset was a large-scale study that analyzed 20,134,421 SNPs and included 472,174 participants aged 40–69 years. The mean TL in the database was calculated using definitive quantitative PCR assays. A thorough quality check and technical adjustments were performed. Additionally, statistical adjustments were made for age to mitigate its impact on TL. The racial composition of the dataset was predominantly European Caucasians. The GWAS summary data from another TL study were obtained from Kessler et al. for replication 22 . This study quantified TL in 418,401 individuals of European ancestry using exome-wide sequencing. Proteomic MR analysis In the MR analysis, we used summary GWAS statistics for plasma proteins as the exposure data and TL as the outcome data. We first selected SNPs that were strongly associated with proteins (p < 5 × 10–8) as IVs. Because the bias in the results may be caused by the high level of linkage disequilibrium (LD) between the instrumental variables, we set the LD between the selected SNPs to > 10,000 kb and the correlation coefficient (r2) < 0.01, to ensure mutual independence of each SNP. In addition, the robustness of the genetic IVs was estimated using R2 and F statistics (R2 = 2 × (1 − EAF) × EAF × beta2; F = R2 (N − 2)/(1 − R2)) 23 to increase power. The F-statistic for each IV in this study exceeded 10. A pQTL was considered a cis-pQTL if the lead SNP was located no more than one million base pairs from the transcription start site of the protein-encoding gene, and a pQTL found beyond this designated region was considered a trans-pQTL 24 . All genetic tools for plasma protein levels were constructed using cis-pQTLs, as the use of cis-pQTLs (pQTL close to protein-coding genes) is more conducive for adherence to the core assumptions of MR 25 , 26 . Information regarding IVs is provided in Supplementary Table S2 . The "TwoSampleMR" package with R language version 4.3.2 was used to perform the MR analysis. If two or more pQTL were available, the MR effect was estimated by inverse variance weighting. If only one pQTL was available, then the MR effect was estimated using the Wald ratio. To confirm the accuracy of the results, we performed sensitivity and heterogeneity tests based on Q statistics. Effect estimates were calculated using MR-Egger (adjusting for the residual correlation between variables), a model that accounts for horizontal pleiotropy. When heterogeneity was present, we chose the inverse variance-weighted (multiplicative random effects) model for the MR analysis. When pleiotropy was present, MR-Presso was used to test for outliers and confounders in the MR analysis. In the discovery cohort, we used the Bonferroni method for multiple correction of p-values, defining p < 1.91 × 10–5 (0.05/2611) as the significance level. In the validation cohort, we defined a nominal p-value < 0.05 as the level of significance. Finally, to examine whether a reverse causality bias existed, we performed a Steiger directionality test to determine whether our MR analyses were significantly affected by reverse causality. Bayesian colocalization analyses We performed Bayesian colocalization analyses by using the "coloc" R package 27 to evaluate whether consistent causal variation (rather than variation driven by linkage disequilibrium) was shared between TL and identified pQTLs. The colocalization analysis was based on five core hypotheses: H0, there was no causal variant for either of the two traits; H1, there was a causal variant for protein only; H2, there was a causal variant for TL only; H3, protein and TL had two different causal variants; and H4, protein and TL shared the same causal variant. Each of these hypotheses (H0, H1, H2, H3, and H4) corresponds to a posterior probability (i.e., PPH0, PPH1, PPH2, PPH3, and PPH4) 28 . We used SNPs within ± 1000 kb of the pQTL for pQTL-GWAS colocalization. If multiple pQTLs were present, a colocalization analysis was conducted separately for each pQTL, and the pQTL with the most robust colocalization evidence was displayed. The prior probabilities are respectively set at p1 = 1e−4, p2 = 1e−4, and p12 = 1e−5. We considered that PPH4 (posterior probability that protein and TL shared the same causal variant) greater than 80% constitutes robust evidence in favor of colocalization 29 , 30 . Summary-data-based Mendelian randomization (SMR) SMR analysis was used as a complementary method to further explore the causal relationship between MR-identified proteins and TL. SMR analysis was conducted using SMR software version 1.3.1. The heterogeneity in the dependent instrument (HEIDI) test was also applied. When PHEIDI > 0.05, the link between protein and TL was not influenced by LD. The Bonferroni correction was applied to adjust the results of multiple testing, setting the threshold of significance for SMR at a p-value of < 2.38 × 10−3 (21 target proteins). Causal effects were considered to be statistically significant and not driven by cascading imbalances when the p-value was < 2.38 × 10−3 and P-HEIDI > 0.05. We categorized the MR-identified proteins of the discovery cohort into three tiers based on results of association with TL. Proteins that passed both the colocalization analysis (PPH4 > 80%) and the SMR analysis (p < 2.38 × 10−3) and HEIDI test (p > 0.05) were defined as Tier 1. Proteins that passed only the colocalization analysis or the HEIDI test were defined as Tier 2. Proteins failed both the colocalization and HEIDI test due to insufficient statistical power or missing data were defined as Tier 3. PPI network, enrichment, and druggability assessment We constructed a PPI network using the STRING database and Cytoscape software for visual representation to probe potential interactions between TL-associated proteins. Biological functions of TL-associated proteins were further investigated by Gene Ontology (GO) enrichment analysis, and the results were visualized using R packages such as "clusterProfiler" and "pathview" 31 . In addition, to explore whether the aforementioned proteins can be used as targets of existing drugs or druggable gene targets, we searched for interactions between these proteins and drugs and further explored the druggability of these proteins using the DrugBank 32 database. Phenome-wide MR analysis (MR-PheWAS) To assess the horizontal pleiotropy and possible side effects of potential target proteins more comprehensively, we performed MR-PheWAS analyses of the Tier 1 proteins as exposures. The outcome data for conducting PheWAS were obtained from the UK Biobank, which tested 28 million SNPs for 1403 disease phenotypes in 408,961 White British participants using the SAIGE platform ( https://www.leelabsg.org/resources) 33 . Considering that disease phenotypes with a sample size of more than 500 cases have higher representativeness and stronger statistical validity, we subjected 783 disease phenotypes obtained from selection (n > 500) (Supplementary Table 8 ) to MR-PheWAS analyses 34 . More detailed information is provided in a previous publication 33 . Bonferroni correction was applied to the p-value to adjust for multiple tests, setting the threshold of significance at p < 0.05/783. Results Proteomic MR identified 22 plasma proteins associated with TL Proteomic MR analysis at the discovery stage revealed that 22 proteins from the two protein databases were significantly associated with TL (p < 1.91 × 10–5) after Bonferroni correction (Supplementary Table S3 , Fig. 2 A). Specifically, we observed 11 proteins for which increased protein abundance was significantly and positively associated with TL, including DAG1, USP8, SPRED2, BET1L, MAX, ATP6V1G2, TREH, CTRL, NFE2, VSNL1, and RALY. Increased protein abundance of 11 proteins was significantly negatively correlated with TL, including in PSMB1, ARPC1B, WBP2, GMPR2, NPPA, APOBR, APOE, ATOX1, IL27, and TCL1A, and YES1. Fig. 2 Summary data from the two-stage MR (discovery and replication) and colocalization analysis on the causal relationship between proteins and TL. (A) Volcano plot of the MR results in the discovery stage between 22 plasma proteins and TL. The tagged proteins passed multiple test corrections (p < 1.91 × 10–5). (B) Bubble plot showing the results of colocalization analysis between plasma proteins and TL. (C) MR analysis of 22 proteins in the discovery and replication datasets. Discussion To our knowledge, this is the first MR study to comprehensively explore the effects of plasma proteome-centric targets of specific proteins on TL. This MR study analyzed the association between plasma proteins and TL, supplemented by colocalization analysis and SMR analyses. In the discovery stage of the proteomic MR study, 22 protein markers associated with TL were identified, of which elevated levels of 11 proteins and reduced levels of another 11 correlated with increased TL. Replication MR validated 20 of 22 candidate proteins. Among these, five proteins (e.g., APOE, SPRED2, MAX, RALY, and PSMB1), were strongly supported by colocalization and passed the SMR and HEIDI tests, with the highest level of evidence of association with TL. Among these, an increased abundance of SPRED2, MAX, and RALY proteins was positively associated with TL, whereas an increased abundance of APOE and PSMB1 proteins was negatively associated with TL. Phenome-wide MR analyses indicated potential target effects, both favorable and unfavorable, and highlighted safety issues regarding the use of target proteins for medicinal purposes. Overall, these findings provided new insights into the biological mechanisms and potential effector targets of TL. Among the candidate proteins identified, some have been suggested to be associated with TL in terms of gene polymorphisms or protein levels. For example, previous GWAS have shown that genetic polymorphisms in APOE, NFE2, SPRED2, MAX, and TCL1A are associated with TL 21 , 35 , 36 , suggesting that the data sources used in the current analysis have good validity. Among them, APOE, SPRED2, and MAX were classified as Tier 1 proteins with the highest level of evidence in this study. Recently, it has been shown that APOE may indirectly regulate telomere length in neural stem cells (NSCs) by inhibiting sirtuin 1(SIRT1) and fibroblast growth factor 2 (FGF2) 37 . Dhillon et al. showed that Apolipoprotein-ε4 (APOE-ε4) carriers have shorter telomeres than non-carriers and that carriers suffer from related diseases such as dementia 38 . However, we found that the two most common variants of APOE subtypes, rs429358 and rs7412, did not appear in our study, which may stem from differences in assay methodology, population structure, and genetic background from previous studies. Interestingly, the SNP rs483082 in this study and APOE-ε4 (encoded by rs429358) signaling showed a strong association in previous studies and together affect Alzheimer's disease-related pathological features, especially in regulating Aβ40/42 levels 39 , 40 . This may be due to their location in the same linkage disequilibrium (LD) region. RALY is an RNA-binding protein 41 that has been found to be a component of the Telomeric repeat-containing RNA(TERRA) interactome in mouse embryonic stem cells 42 . TERRA is a long-stranded, non-coding RNA transcribed from telomeres that plays a key role in maintaining telomere length and stability 43 . Recent studies have shown that RALY depletion leads to reduced levels of TERRA, disrupting its localization at telomeres and eventually causing telomere damage 44 . These findings are consistent with the results of our study. Our study further confirmed the causal association of these genes with TL at the protein level and extended the evidence for the genetic association of downstream mechanisms. Our study identified several novel TL protein biomarkers. For example, the proteasome subunit beta type-1 (PSMB1) has the strongest evidence (Tier 1). Our study suggests that PSMB1 has a potential inhibitory effect on TL. However, no evidence on the association between PSMB1 and TL has been obtained from observational epidemiological and experimental studies. PSMB1 is the β6 subunit in the 20S proteasome and serves as a checkpoint for the assembly of proteasome dimerization 45 , 46 . Moreover, PSMB1 plays a critical role in maintaining protein homeostasis and is involved in various biological processes. Previous studies have shown that PSMB1 promotes proteasome-dependent degradation of IκB kinase ε (IKK-ε) and inhibits interferon signaling 47 . Recently, new evidence suggests that PSMB1 acts as a negative regulator of the Rankl-induced NF-κB pathway, targeting IKK-β to inhibit NF-κB activation 48 . There is evidence for an association between NF-κB activation and telomerase reverse transcriptase (TERT) expression. For example, transcriptional up-regulation of TERT was selectively induced by activation of NF-κB in endosomal smooth muscle cells 49 , and it was observed in mouse tissues that NF-κB may contribute to the activation of TERT expression 50 , which is essential for the maintenance of TL 50 . Therefore, it is reasonable to hypothesize that PSMB1 may reduce TERT expression by targeting the inhibition of NF-κB activity, ultimately leading to telomere length shortening. However, further epidemiological and experimental studies are required to confirm these findings. One advantage of our study is that it is the first large-scale study to jointly apply three methods (MR, colocalization, and SMR) to investigate the causal effects between TL and protein biomarkers. The MR design reduces the bias from confounding factors and reverses causality, thereby enhancing the reliability of the causal inference. Additionally, the colocalization analysis has been shown to be an effective method for reducing biases caused by potential LD. The large sample size of the GWAS significantly enhanced the statistical robustness of our analysis. In addition, the consistency of the MR results between the discovery and replication datasets for TL provides strong evidence supporting our conclusions. Our study had some limitations. First, we only included cis-pQTL, which is closer to the protein-coding gene, as a genetic IV for the MR analysis. Therefore, we cannot exclude other proteins that have significant trans-pQTL but were not included in the analysis due to the screening conditions. Second, strict evidence grading standards and significance threshold screening methods may underestimate the persuasive power of certain TL-associated proteins, resulting in false negative results. For example, the absence of complete summary data precluded the possibility of conducting colocalization and SMR analyses on IL27. Furthermore, because our GWAS dataset exclusively included European populations, the applicability of our findings to different ethnicities and groups may be limited 51 . Therefore, a larger cross-race meta-analysis is required to extend the identification of TL-associated causal variants to other ethnic groups. Finally, MR only provides insights into causal associations and its direction, because genetic variation reflects the lifelong effects of changes in protein levels on TL rather than making quantitative estimates 52 . Therefore, future studies are necessary to further validate the relationship between identified proteins and TL and to clarify the potential biological mechanisms through population-based studies as well as in vivo and in vitro experiments. Conclusions In this study, we identified plasma proteins associated with TL through comprehensive MR analysis, and these telomere-associated genetic determinants represent potential therapeutic targets for aging-related diseases and deepen the understanding of the mechanisms of telomere biology and its relationship to aging. Data availability All GWAS data used in this study are publicly available, and the GWAS summary data for TL are available from the MRC-IEU OpenGWAS database ( https://gwas.mrcieu.aco/ ) and GWAS Catalog ( https://www.ebi.ac.uk/gwas/ ). Summary data for plasma proteins are available from UKB-PPP ( https://www.ukbiobank.ac ) and deCODE ( https://www.decode.com/summarydata/ ). References Acknowledgements We thank all the participants in the UK Biobanking Study, the deCODE project, the two GWASs of telomere length, and the researchers who made the GWAS data publicly available. Author information Authors and Affiliations Department of Clinical Laboratory, North China University of Science and Technology Affiliated Tangshan Maternal and Child Health Care Hospital, Tangshan, China Jiaxuan Zhao, Kun Yang, Yunfei Lu, Linfeng Zhou, Haoran Fu & Jinghua Wu Key Laboratory of Molecular Medicine for Abnormal Development and Related Diseases in Tangshan City, Tangshan, China Jiaxuan Zhao, Kun Yang, Yunfei Lu, Linfeng Zhou, Haoran Fu & Jinghua Wu The 982th Hospital of the People’s Liberation Army Joint Logistics Support Force, Tangshan, China Jingbo Feng Supplementary Information Rights and permissions Open Access This article is licensed under a Creative Commons Attribution 4.0 International License, which permits use, sharing, adaptation, distribution and reproduction in any medium or format, as long as you give appropriate credit to the original author(s) and the source, provide a link to the Creative Commons licence, and indicate if changes were made. The images or other third party material in this article are included in the article's Creative Commons licence, unless indicated otherwise in a credit line to the material. If material is not included in the article's Creative Commons licence and your intended use is not permitted by statutory regulation or exceeds the permitted use, you will need to obtain permission directly from the copyright holder. To view a copy of this licence, visit http://creativecommons.org/licenses/by/4.0/ .
Decode Health Frequently Asked Questions (FAQ)
When was Decode Health founded?
Decode Health was founded in 2019.
Where is Decode Health's headquarters?
Decode Health's headquarters is located at 111 10th Avenue South, Nashville.
What is Decode Health's latest funding round?
Decode Health's latest funding round is Spinoff / Spinout.
Who are the investors of Decode Health?
Investors of Decode Health include IQuity Labs, Small Business Innovation Research Awards and SwitchPoint Ventures.
Who are Decode Health's competitors?
Competitors of Decode Health include Tempus, Predicta Med, Prealize, ClosedLoop, Jvion and 7 more.
What products does Decode Health offer?
Decode Health's products include Decode Health Analytics Platform.
Who are Decode Health's customers?
Customers of Decode Health include Quest Diagnostics and Beckton Dickinson.
Loading...
Compare Decode Health to Competitors
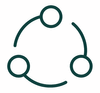
Atropos Health specializes in transforming healthcare data into actionable evidence for the healthcare and life sciences sectors. The company offers solutions that support clinical decisions, optimize health systems, and accelerate clinical research by leveraging a vast network of patient records and analytics tools. Atropos Health primarily serves the healthcare industry, including health systems and life science companies, by providing insights for formulary design, quality improvement programs, and clinical trial emulations. It was founded in 2019 and is based in New York, New York.
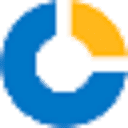
ClosedLoop operates as a healthcare data science platform. It enables providers, payers, and value-based care organizations to make accurate, explainable, and actionable predictions of individual-level health risks, outcomes, and costs. It was formerly known as Deep Health Technologies. The company was founded in 2017 and is based in Austin, Texas.
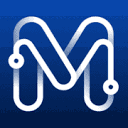
Medicom specializes in healthcare interoperability and operates within the health information technology sector. The company offers a federated health information network that aggregates and facilitates the exchange of health data through a single interface, enabling healthcare providers to access and share clinical records. Medicom's primary customer segments include the healthcare industry, government health agencies, and life sciences organizations. It was founded in 2015 and is based in Raleigh, North Carolina.
Perthera operates as a healthcare artificial intelligence company focusing on advancing precision medicine. The company offers an artificial intelligence (AI) enabled platform utilizing patient history and multi-omic testing results to match cancer patients with ranked therapy options, and accelerates clinical and therapeutic innovation. It was formerly known as Personalized Cancer Therapy. It primarily serves the healthcare industry in the oncology sector. It was founded in 2012 and is based in McLean, Virginia.
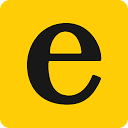
Evidation focuses on harnessing real-world health data to measure and improve health outcomes. The company offers a digital health measurement and engagement platform that utilizes data science and machine learning to provide health guidance, treatments, and tools. Evidation primarily serves life sciences companies, government bodies, and academic institutions. It was founded in 2012 and is based in San Mateo, California.

Datavant operates as a company focused on health data connectivity in the healthcare industry. It offers services that securely and compliantly connect patient-level health data, providing solutions for data and analytics, life sciences, providers, government agencies, research networks, and health plans. Its primary customers are organizations within the healthcare sector. The company was founded in 2017 and is based in San Francisco, California.
Loading...