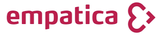
Empatica
Founded Year
2011Stage
Series B - II | AliveTotal Raised
$32.19MMosaic Score The Mosaic Score is an algorithm that measures the overall financial health and market potential of private companies.
-74 points in the past 30 days
About Empatica
Empatica is an affective computing company that specializes in medical quality sensing and human data analytics within the health monitoring and research sector. The company offers FDA-cleared wearable devices and a health monitoring platform that provides accurate, continuous health insights for researchers and clinicians, collected in real-world settings. Empatica's technology is utilized by pharmaceutical companies to monitor treatment impacts and develop novel digital biomarkers. Empatica was formerly known as Physiio International,Inc.. It was founded in 2011 and is based in Cambridge, Massachusetts.
Loading...
Empatica's Product Videos
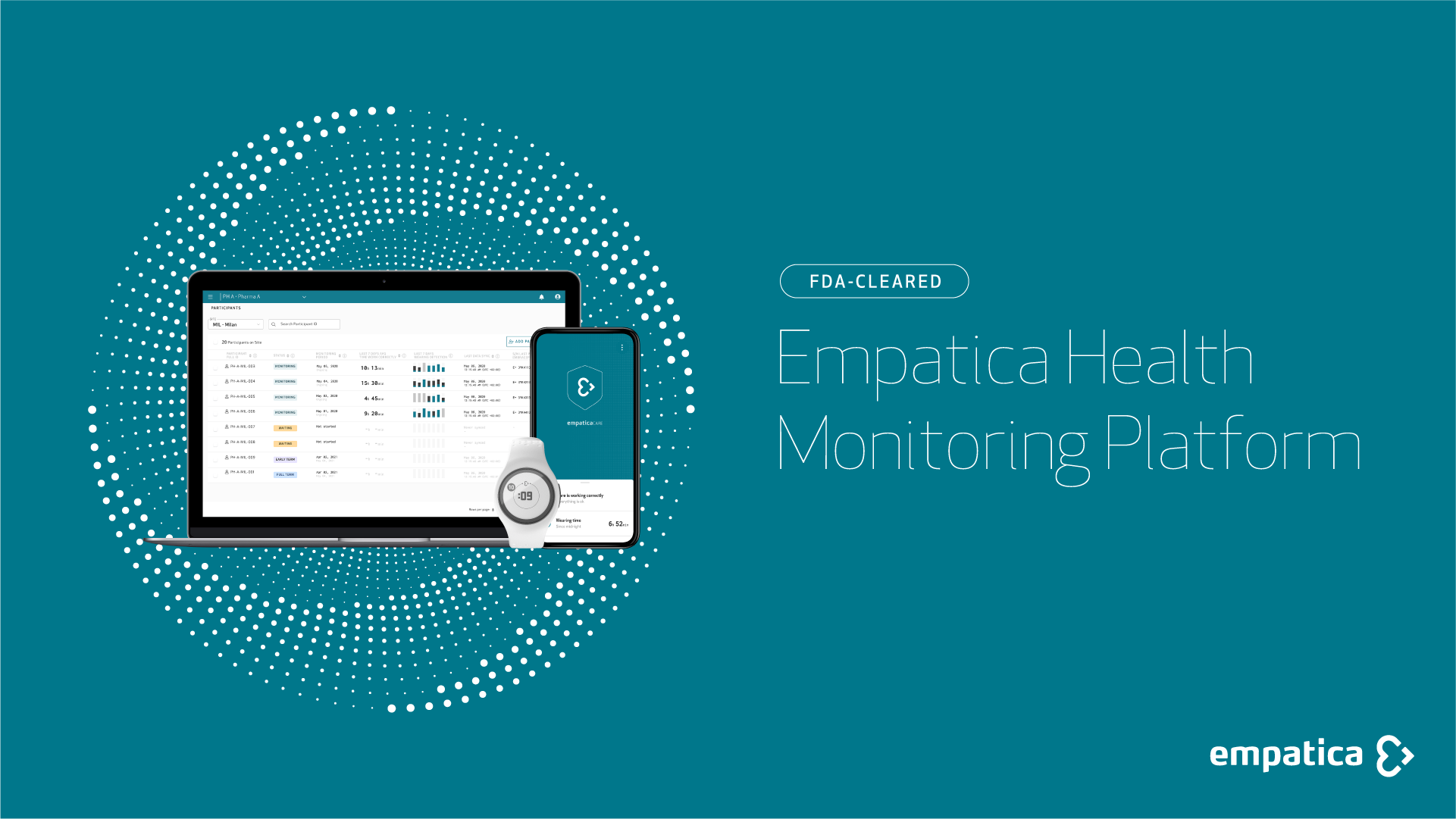
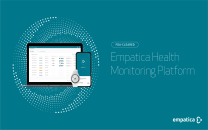
ESPs containing Empatica
The ESP matrix leverages data and analyst insight to identify and rank leading companies in a given technology landscape.
The digital biomarker monitoring market provides technology solutions that capture and analyze digital health data to assess and predict health outcomes. Utilizing devices and software that integrate into patients’ daily lives, these solutions monitor various physiological and behavioral indicators that serve as biomarkers for health conditions. This market includes mobile apps that track activity…
Empatica named as Challenger among 15 other companies, including Whoop, Huma, and AliveCor.
Empatica's Products & Differentiators
Empatica Health Monitoring Platform
The Empatica Health Monitoring Platform is an FDA-cleared modular remote health monitoring platform. It provides healthcare and research professionals with a full-stack product suite, consisting of Empatica’s medical-grade EmbracePlus hardware, software, digital biomarkers and Cloud. The platform enables the continuous collection, streaming and storage of physiological signals and digital biomarkers. Professionals can use the Empatica Health Monitoring Platform for to collect data from small and large-scale scientific and clinical research studies, monitor patient compliance, set up their studies and manage their participants.
Loading...
Research containing Empatica
Get data-driven expert analysis from the CB Insights Intelligence Unit.
CB Insights Intelligence Analysts have mentioned Empatica in 5 CB Insights research briefs, most recently on Aug 21, 2024.

Aug 21, 2024
The clinical trials tech market map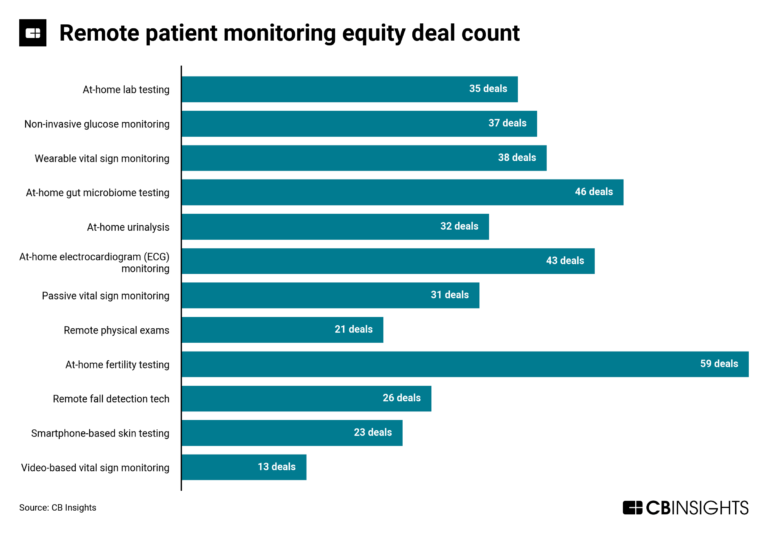
Aug 15, 2023
The remote patient monitoring market mapExpert Collections containing Empatica
Expert Collections are analyst-curated lists that highlight the companies you need to know in the most important technology spaces.
Empatica is included in 5 Expert Collections, including Artificial Intelligence.
Artificial Intelligence
14,767 items
Companies developing artificial intelligence solutions, including cross-industry applications, industry-specific products, and AI infrastructure solutions.
Smart Home & Consumer Electronics
1,234 items
This Collection includes companies developing smart home devices, wearables, home electronics, and other consumer electronics.
Digital Health
11,074 items
The digital health collection includes vendors developing software, platforms, sensor & robotic hardware, health data infrastructure, and tech-enabled services in healthcare. The list excludes pureplay pharma/biopharma, sequencing instruments, gene editing, and assistive tech.
Telehealth
2,918 items
Companies developing, offering, or using electronic and telecommunication technologies to facilitate the delivery of health & wellness services from a distance. *Columns updated as regularly as possible; priority given to companies with the most and/or most recent funding.
Precision Medicine Tech Market Map
160 items
This CB Insights Tech Market Map highlights 160 precision medicine companies that are addressing 9 distinct technology priorities that pharmaceutical companies and healthcare providers face.
Empatica Patents
Empatica has filed 15 patents.
The 3 most popular patent topics include:
- electrical engineering
- analog circuits
- capacitors
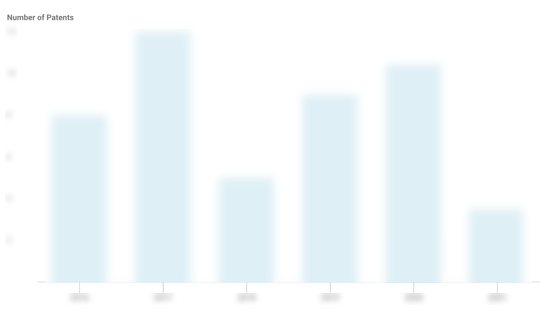
Application Date | Grant Date | Title | Related Topics | Status |
---|---|---|---|---|
5/5/2020 | 3/5/2024 | Radio electronics, Noise (electronics), Telecommunication theory, Communication circuits, Broadcast engineering | Grant |
Application Date | 5/5/2020 |
---|---|
Grant Date | 3/5/2024 |
Title | |
Related Topics | Radio electronics, Noise (electronics), Telecommunication theory, Communication circuits, Broadcast engineering |
Status | Grant |
Latest Empatica News
Sep 23, 2024
December 21, 2023 . Toward Physiological Detection of a “Just-Right” Challenge Level for Motor Learning in Immersive Virtual Reality: Protocol for a Cross-Sectional Study Toward Physiological Detection of a “Just-Right” Challenge Level for Motor Learning in Immersive Virtual Reality: Protocol for a Cross-Sectional Study Authors of this article: 2Centre de Recherche du Centre Hospitalier Universitaire Sainte-Justine, Montréal, QC, Canada 3Department of Physical Activity Sciences, Faculty of Sciences, Université du Québec à Montréal, Montréal, QC, Canada Corresponding Author: Montréal, QC, H1T 1E1 Abstract Background: Motor learning, a primary goal of pediatric rehabilitation, is facilitated when tasks are presented at a “just-right” challenge level—at the edge of the child’s current abilities, yet attainable enough to motivate the child in persistent efforts for success. Immersive virtual reality (VR) may be ideally suited for “just-right” task challenges because it enables precise adjustments of task parameters in motivating environments. Rehabilitation-specific VR tasks often use dynamic difficulty algorithms based on task performance to personalize task difficulty. However, these approaches do not consider relevant cognitive processes that could also impact “just-right” challenges, such as attention and engagement. Objective physiological measurement of these cognitive processes using wearable sensors could support their integration within “just-right” challenge detection and prediction algorithms. As a first step, it is important to explore relationships between objectively and subjectively measured psychophysiological states at progressively challenging task difficulty levels. Objective: This study aims to (1) evaluate the performance of wearable sensors in a novel movement-based motor learning immersive VR task; (2) evaluate changes in physiological data at 3 task difficulty levels; and (3) explore the relationship between physiological data, task performance, and self-reported cognitive processes at each task difficulty level. Methods: This study uses the within-participant experimental design. Typically developing children and youth aged 8-16 years will be recruited to take part in a single 90-minute data collection session. Physiological sensors include electrodermal activity, heart rate, electroencephalography, and eye-tracking. After collecting physiological data at rest, participants will play a seated unimanual immersive VR task involving bouncing a virtual ball on a virtual racket. They will first play for 3 minutes at a predefined medium level of difficulty to determine their baseline ability level and then at a personalized choice of 3 progressive difficulty levels of 3 minutes each. Following each 3-minute session, participants will complete a short Likert-scale questionnaire evaluating engagement, attention, cognitive workload, physical effort, self-efficacy, and motivation. Data loss and data quality will be calculated for each sensor. Repeated-measures ANOVAs will evaluate changes in physiological response at each difficulty level. Correlation analyses will determine individual relationships between task performance, physiological data, and self-reported data at each difficulty level. Results: Research ethics board approval has been obtained, and data collection is underway. Data collection was conducted on December 12, 2023, and April 12, 2024, with a total of 15 typically developing children. Data analysis has been completed, and results are expected to be published in the fall of 2024. Conclusions: Wearable sensors may provide insights into the physiological effects of immersive VR task interaction at progressive difficulty levels in children and youth. Understanding the relationship between physiological and self-reported cognitive processes is a first step in better identifying and predicting “just-right” task challenges during immersive VR motor learning interventions. International Registered Report Identifier (IRRID): DERR1-10.2196/55730 JMIR Res Protoc 2024;13:e55730 Figure 3. Difficulty selection and progression depending on baseline evaluation. After each 3-minute play session, the participant removes the HMD and answers a study-specific questionnaire asking them to rate agreement with 7 statements (1 statement per construct) about their engagement, attention or focus, cognitive workload, physical effort, self-efficacy, and motivation. Statements are presented on a 7-item Likert scale with anchors on “strongly disagree” and “strongly agree.” First, engagement—2 questions inspired by the Independent Television Commission-Sense Of Presence Inventory [ 27 ] whose scores are averaged as (1) I enjoyed myself during this level and (2) I felt involved in the displayed environment. Second, attention or focus—I am completely focused on the task at hand (question derived from the Flow Short Scale [ 28 ]). Third, cognitive workload—during the task I did a lot of thinking and figuring out (questions derived from the revised National Aeronautics and Space Administration [NASA] Task Load Index [TLX] for children [ 29 ]). Fourth, physical effort—during the task I had to do a lot of physical effort (question derived from the revised NASA TLX for children [ 29 ]). Fifth, self-efficacy—I think I can do well at a more difficult level. Finally, motivation—I wanted to work harder to improve my performance or I tried hard to improve my performance (question derived from the Pediatric Motivation Scale [ 30 ]) The resting period between each 3-minute play session is between 3 and 5 minutes, and participants can request a longer break as needed. At the end of the session, participants complete the Child Simulator Sickness Questionnaire [ 31 ]. Participants will receive a CAD $25 (US $1=CAD $1.37) gift card as a token of appreciation for their participation in the study. Data Collection Instruments Task Performance Participants will wear a Pico 4 Enterprise HMD. Performance data are collected within the Unity task—for each trial, the position of the paddle when the ball hits it and the maximum elevation of the ball after each hit is captured. The number of consecutive hits is calculated. To be considered as a valid bounce the ball must reach a height of at least 15 cm. Task performance is defined as the ratio of error over the total number of bounces attempted (number of errors divided by [number of bounces + number of errors]) during the 3-minute trial. EEG Analysis A Kaptics (Corporation) EEG with 12 Ag/AgCl dry electrodes is integrated into the Pico4 headgear. The electrodes are placed in the configuration—Fp1, Fp2, AFz (bias), F3, Fz, F4, C3, Cz (reference), C4, O1, Oz, O2. The Kaptics custom-developed application computes the psychophysiological metrics of (1) mental engagement level, measured with the engagement index, which is calculated through beta and alpha bands, based on the work of Coelli et al [ 32 ]; (2) cognitive workload, which is calculated through changes in theta and alpha bands, based on the work of Di Flumeri et al [ 33 ] and Zammouri et al [ 34 ]; and (3) concentration, which is measured through changes in beta bands, based on the work of Lim et al [ 35 ]. Eye-Tracking The Pico Neo 4 Enterprise has an integrated Tobii eye tracker. Oculometry correlates with attention to a task [ 36 ]. The average time per trial of eye pursuit of the ball will be used as a metric of focused attention. Eye pursuit will be computed as the amount of time when performing the VR task, during which the direction of the eyes intersects with the areas of interest (volumes englobing respectively the ball and the paddle). A lower blink rate will be indicative of a higher focused attention [ 36 ]. Movement Quantity The continuous position of the virtual paddle in the virtual environment is saved at a frame rate of 60 Hz. From the consecutive 3D positions recorded, the total amount of displacement will be computed to get the total amount of motion during the virtual task. EDA and HR Participants will wear an Empatica E4 sensor equipped with a photoplethysmography sensor and an EDA sensor. EDA, a recognized marker of arousal [ 37 ], will be computed as the average number of peaks per minute for each 3-minute play period using pyEDA, an open-source Python toolkit (Python Software Foundation) [ 38 ]. HR is also a proxy for arousal [ 37 ]. HR in beats per minute will be averaged over each gameplay. The changes in the level of arousal will be computed to determine the intensity of the physiological response. With an optimal level of engagement, arousal should be average [ 37 ]. If arousal is too high it can be interpreted as a sign of frustration rather than engagement. Upper Extremity Movement Quality As an exploratory measure, participants will wear XSens DOT inertial measurement units on the active forearm and on the chest to capture upper limb movement smoothness. Movement smoothness is measured through acceleration data. Less smooth movements are characterized by fluctuations in velocity, causing local maxima in the velocity profile. It is classified as an acceleration metric since the calculation of this metric is in the acceleration domain [ 39 ]. The lower the number of local maxima recorded the smoother the movement is. The average number of local maxima per minute will be computed as an exploratory measure to look for differences in movement at the different levels of difficulty. Data Processing Empatica data are stored in the smartwatch during the experiment and then downloaded to a computer for analysis. EDA data will be filtered using a low-pass Butterworth filter (frequency of 1 Hz and order of 6). EEG signals processed by Kaptics are filtered using a band-pass filter between 0.5 and 45 Hz and then zero-mean normalized. Kaptics uses the subspace reconstruction method to remove motion artifacts [ 40 ]. Analyses Overview Quantitative statistical analyses will be performed using SPSS (IBM Corp) for ANOVA, correlations and graphical output and R (R Foundation for Statistical Computing) for the usage of the repeated measure correlation (rmcorr) package [ 26 ]. Confidence level will be set at 95%. Descriptive statistics will be presented as counts, means, and standard differences by level of difficulty. Demographic categorial data will be presented as means and standard differences by age, gender, and Gross Motor Function Classification System level. Objective 1 The aim is to evaluate the performance of the physiological sensors in children undertaking a new motor learning task in immersive VR. Identify Data Loss The acceptable data loss threshold per measure will be set as 10% of the total data collection time for each of the 3 gameplay sessions. The expected output of data differs by sensor according to frequency. The amount of recorded data, for each 3-minute level, over expected output will be computed to measure the percentage of data loss. EDA: Empatica frequency is 4 Hz HR: Empatica HR value frequency is 1 Hz Eye-tracking: Data will be collected at a fixed frequency (60 Hz). When the HMD is unable to accurately detect the eyes of the participants, the values are reported as 0. Data Quality For each 3-minute level, the amount of aberrant data will be computed for each sensor. The percentage of aberrant data over the total amount of collected data will then be computed. First, the quality of EDA data is assessed following the two criteria of [ 41 ] (1) the total range of valid EDA data is 0.05-60 µS (data outside this range will be considered aberrant) and (2) an EDA change of more than ±10 µS/s between any 2 consecutive values is considered aberrant. Second, HR—the minimal valid value of HR data is 60 beats per minute [ 42 ], while the estimated maximum valid value is 194 beats per minute [ 43 ]. Data outside this threshold will be considered aberrant. Changes in HR between any 2 consecutive values superior to ±3 beats per second will be considered aberrant [ 44 ]. Objective 2 Evaluate changes in physiological data at 3 immersive VR task difficulty levels. To examine intraindividual changes in averaged physiological data at baseline and the 3 difficulty levels, a repeated measure ANOVA will be performed for each dependent variable, with the level of difficulty as the within-subject factor. Sensitivity analysis will be done using the Friedman test, due to the small sample size. Eta squared will be reported as the measure of effect size. Objective 3 The aim is to explore the relationship between physiological data, task performance, and self-reported measures of engagement, cognitive workload, physical effort, and attention or focus at each task difficulty level. To determine the intraindividual relationship between physiological data and self-reported data, the Pearson product-moment correlation, and the Spearman rank correlation will be performed, to explore linear and monotonic relationships. The complete correlation matrix will be reported (with correlation coefficient as effect sizes and P values). At each difficulty level, correlations will be explored between (1) the level of engagement computed from the EEG data and the averaged self-reported score of engagement, (2) the level of attention computed from the EEG data and the self-reported score of attention, (3) the level of attention computed from the eye tracking data (time of pursuit and average blink rate) and the self-reported score of attention, (4) the level of cognitive workload computed from the EEG data and the self-reported score of cognitive workload, and (5) the average amount of movement of the virtual paddle and the self-reported score of physical effort. Testing to evaluate parallel slopes between conditions will be undertaken [ 45 ]. If parallel slopes are identified, a repeated measure correlation (rmcorr) will be performed [ 26 ]. The rmcorr method can handle repeated measures data without violating independence assumptions or averaging data. It is ideally suited to assess association in intraindividual relationships between paired measures. Visual analysis [ 46 ], as well as statistical inference (regression coefficients and P values) will be reported. We will report visual analyses, as well as statistical inference (regression coefficients and P values) results. Results Study recruitment is underway. The Centre hospitalier universitaire Sainte-Justine research ethics board approved this work (2022-3881) in the spring of 2023. Data collection was conducted on December 12, 2023, and April 12, 2024, with a total of 15 typically developing children. Data analysis has been completed, and results are expected to be published in the fall of 2024. Discussion Principal Findings Targeting “just-right” task challenges during rehabilitation interventions is anchored in evidence-based motor learning principles [ 3 ]. Wearable sensors can enable objective measurement of psychophysiological states related to difficulty progression during motor skill learning. A better understanding of wearable sensor task performance during movement-based tasks in children is required [ 24 ]. The current state of knowledge on wearable sensor use in pediatric rehabilitation is limited to inertial measurement units and accelerometers [ 19 ]. Very few studies have explored the potential of wearable physiological sensors to understand children’s engagement during VR-based interactions [ 22 ]. This study will evaluate relationships between physiological data and children’s self-reports during the practice of a novel motor learning task in immersive VR at different task difficulty levels. Findings from this pilot study will inform subsequent work, which could include collecting physiological and self-report data with a child with a motor impairment who progresses at their own pace through task difficulty levels in immersive VR and asking children to self-identify when they perceive the challenge to be “just-right.” If objective and subjective data are correlated, this training data could be used to build a machine learning model to predict the “just-right” challenge based on the combination of thresholds of different variables, using the subjective self-report of the “just-right” challenge to correctly label the corresponding physiological data. As proposed by Houzangbe et al [ 18 ], combining the success rate of a task with psychophysiological levels of engagement, arousal, cognitive workload, and attention could lead to the objective identification of conditions necessary to reach personalized “just-right” challenges. In the longer term, embedding this model within immersive VR motor learning tasks may enable real-time decision-making about task difficulty level to achieve and maintain “just-right” task challenges. Subsequent work can also compare different types of VR tasks to assess the reproducibility of the results and their generalization. Potential Limitations The small sample size of this pilot study limits the scope of the conclusions. Results will inform the calculation of the effect size required to power subsequent data collection. Using commercial physiological wearable sensors may lead to more compromised data quality as compared to medical-grade equipment. This limitation is balanced by cost and accessibility benefits. Choosing not to counterbalance task difficulty presentation introduces potential learning or fatigue effects. Using short house-made motivation and focus questionnaires is required as longer validated questionnaires are impractical following short gameplay sessions. Conclusions This is the first step in a program of research exploring factors influencing children’s user experiences during motor skill learning in immersive VR. Immersive VR hardware and software are rapidly developing and lowering in cost, increasing their potential as an accessible telerehabilitation modality. If the difficulty of immersive VR tasks can be adapted to “just-right” challenges in the absence of therapeutic decision-making, they may be evidence-based, accessible, and personalized telerehabilitation interventions. Being able to identify, quantify, and predict “just-right” challenges can contribute to a future of precision rehabilitation [ 19 ] where VR, physiological sensors, and AI models can provide personalized interventions in clinic and home-based contexts. Acknowledgments Authors would like to thank the Regroupement INTER (Ingénieries de technologies interactives en readaptation), the FRQNT (Fonds de recherche du Québec—Nature et technologies), and the REPAR (Réseau Provincial de Recherche en Adaptation-Réadaptation) for funding this work. DEL is supported by a J2 Scholar award from the Fonds de Recherche du Québec—Santé. Data Availability The datasets generated and analyzed during this study are available from the corresponding author upon reasonable request. Authors' Contributions All authors contributed to the design of the study protocol. SH wrote the first draft of the paper, and all authors contributed to the subsequent versions. SH developed and integrated the data collection tools from the virtual reality software. Conflicts of Interest References Fusco A, Tieri G. Challenges and perspectives for clinical applications of immersive and non-immersive virtual reality. J Clin Med. 2022;11(15):4540. [ FREE Full text ] [ CrossRef ] [ Medline ] Weiss PL, Keshner EA, Levin MF, editors. Virtual Reality for Physical and Motor Rehabilitation. New York, NY. Springer; 2014. Levac DE, Sveistrup H. Motor learning and virtual reality. In: Weiss PL, Keshner EA, Levin MF, editors. Virtual Reality for Physical and Motor Rehabilitation. New York, NY. Springer; 2014:25-46. Holt CJ, McKay CD, Truong LK, Le CY, Gross DP, Whittaker JL. Sticking to it: a scoping review of adherence to exercise therapy interventions in children and adolescents with musculoskeletal conditions. J Orthop Sports Phys Ther. 2020;50(9):503-515. [ CrossRef ] [ Medline ] Demers M, Fung K, Subramanian SK, Lemay M, Robert MT. Integration of motor learning principles into virtual reality interventions for individuals with cerebral palsy: systematic review. JMIR Serious Games. 2021;9(2):e23822. [ FREE Full text ] [ CrossRef ] [ Medline ] Vieira C, da Silva Pais-Vieira CF, Novais J, Perrotta A. Serious game design and clinical improvement in physical rehabilitation: systematic review. JMIR Serious Games. 2021;9(3):e20066. [ FREE Full text ] [ CrossRef ] [ Medline ] Villani D, Riva F, Riva G. New technologies for relaxation: the role of presence. Int J Stress Manag. 2007;14(3):260-274. [ CrossRef ] Riva G, Mantovani F, Gaggioli A. Presence and rehabilitation: toward second-generation virtual reality applications in neuropsychology. J Neuroeng Rehabil. 2004;1(1):9. [ FREE Full text ] [ CrossRef ] [ Medline ] Kaimara P, Oikonomou A, Deliyannis I. Could virtual reality applications pose real risks to children and adolescents? A systematic review of ethical issues and concerns. Virtual Real. 2022;26(2):697-735. [ FREE Full text ] [ CrossRef ] [ Medline ] Poulsen AA, Rodger S, Ziviani JM. Understanding children's motivation from a self‐determination theoretical perspective: implications for practice. Aus Occup Therapy J. May 26, 2006;53(2):78-86. [ CrossRef ] Guadagnoli MA, Lee TD. Challenge point: a framework for conceptualizing the effects of various practice conditions in motor learning. J Mot Behav. 2004;36(2):212-224. [ CrossRef ] [ Medline ] Ashouri S, Letafatkar A, Thomas AC, Yaali R, Kalantari M. The challenge point framework to improve stepping reaction and balance in children with hemiplegic cerebral palsy: a case series study. J Pediatr Rehabil Med. 2023;16(1):37-48. [ CrossRef ] [ Medline ] Hodges NJ, Lohse KR. An extended challenge-based framework for practice design in sports coaching. J Sports Sci. 2022;40(7):754-768. [ CrossRef ] [ Medline ] Chiarello LA, Palisano RJ, Avery L, Hanna S, On Track Study Team. Longitudinal trajectories and reference percentiles for participation in family and recreational activities of children with cerebral palsy. Phys Occup Ther Pediatr. 2021;41(1):18-37. [ CrossRef ] [ Medline ] Valencia Y, Majin J, Guzmán D, Londoño J. Dynamic difficulty adjustment in virtual reality applications for upper limb rehabilitation. 2018. Presented at: IEEE 2nd Colombian Conference on Robotics and Automation (CCRA); 2018 November 01-03; Barranquilla, Colombia. Huber T, Mertes S, Rangelova S, Flutura S, André E. Dynamic difficulty adjustment in virtual reality exergames through experience-driven procedural content generation. 2021. Presented at: IEEE Symposium Series on Computational Intelligence (SSCI); December 5-7, 2021:1-8; Orlando, FL. Browne T. Biofeedback and neurofeedback. In: Friedman HS, editor. Encyclopedia of Mental Health (Second Edition). Oxford. Academic Press; 2016:170-177. Houzangbe S, Zejli Y, Lemay M, Levac D. Quantifying individual and contextual factors that contribute to just-right challenge in an immersive virtual reality pediatric rehabilitation task: a protocol. 2023. Presented at: World Conference of the International Society for Virtual Rehabilitation (WCISVR); 2023 July 1; Montréal, Canada. Lang CE, Barth J, Holleran CL, Konrad JD, Bland MD. Implementation of wearable sensing technology for movement: pushing forward into the routine physical rehabilitation care field. Sensors (Basel). 2020;20(20):5744. [ FREE Full text ] [ CrossRef ] [ Medline ] Lobo MA, Hall ML, Greenspan B, Rohloff P, Prosser LA, Smith BA. Wearables for pediatric rehabilitation: how to optimally design and use products to meet the needs of users. Phys Ther. 2019;99(6):647-657. [ FREE Full text ] [ CrossRef ] [ Medline ] Louie DR, Bird M, Menon C, Eng JJ. Perspectives on the prospective development of stroke-specific lower extremity wearable monitoring technology: a qualitative focus group study with physical therapists and individuals with stroke. J Neuroeng Rehabil. 2020;17(1):31. [ FREE Full text ] [ CrossRef ] [ Medline ] Apicella A, Arpaia P, Giugliano S, Mastrati G, Moccaldi N. High-wearable EEG-based transducer for engagement detection in pediatric rehabilitation. Brain Comput Interfaces. Dec 16, 2021;9(3):129-139. [ CrossRef ] Vaughn J, Gollarahalli S, Shaw RJ, Docherty S, Yang Q, Malhotra C, et al. Mobile health technology for pediatric symptom monitoring: a feasibility study. Nurs Res. 2020;69(2):142-148. [ FREE Full text ] [ CrossRef ] [ Medline ] Behere SP, Janson CM. Smart wearables in pediatric heart health. J Pediatr. 2023;253:1-7. [ CrossRef ] [ Medline ] Freiwald JP, Göbel Y, Mostajeran F, Steinicke F. The cybersickness susceptibility questionnaire: predicting virtual reality tolerance. 2020. Presented at: Proceedings of Mensch und Computer; September 6, 2020:115-118; Magdeburg, Germany. Bakdash JZ, Marusich LR. Repeated measures correlation. Front Psychol. 2017;8:456. [ CrossRef ] [ Medline ] Lessiter J, Freeman J, Keogh E, Davidoff J. A cross-media presence questionnaire: the ITC-sense of presence inventory. Presence: Teleoperators Virtual Environ. 2001;10(3):282-297. [ CrossRef ] Martin AJ, Jackson SA. Brief approaches to assessing task absorption and enhanced subjective experience: examining ‘short’ and ‘core’ flow in diverse performance domains. Motiv Emot. 2008;32(3):141-157. [ CrossRef ] Laurie-Rose C, Curtindale LM, Frey M. Measuring sustained attention and perceived workload. Hum Factors. Feb 2017;59(1):76-90. [ CrossRef ] [ Medline ] Tatla SK, Jarus T, Virji-Babul N, Holsti L. The development of the Pediatric Motivation Scale for rehabilitation. Can J Occup Ther. Apr 2015;82(2):93-105. [ CrossRef ] [ Medline ] Hoeft R, Vogel J, Bowers C. Kids get sick too: a proposed child simulator sickness questionnaire. Proc Hum Factors Ergon Soc Annu Meet. 2016;47(20):2137-2141. [ CrossRef ] Coelli S, Sclocco R, Barbieri R, Reni G, Zucca C, Bianchi A. EEG-based index for engagement level monitoring during sustained attention. Annu Int Conf IEEE Eng Med Biol Soc. 2015;2015:1512-1515. [ CrossRef ] [ Medline ] Di Flumeri G, Borghini G, Aricò P, Sciaraffa N, Lanzi P, Pozzi S, et al. EEG-based mental workload neurometric to evaluate the impact of different traffic and road conditions in real driving settings. Front Hum Neurosci. 2018;12:509. [ FREE Full text ] [ CrossRef ] [ Medline ] Zammouri A, Chraa-Mesbahi S, Moussa AA, Zerouali S, Sahnoun M, Tairi H, et al. Brain waves-based index for workload estimation and mental effort engagement recognition. J Phys Conf Ser. 2017;904(1):012008. [ CrossRef ] Lim S, Yeo M, Yoon G. Comparison between concentration and immersion based on EEG analysis. Sensors (Basel). 2019;19(7):1669. [ FREE Full text ] [ CrossRef ] [ Medline ] Adhanom IB, MacNeilage P, Folmer E. Eye tracking in virtual reality: a broad review of applications and challenges. Virtual Real. 2023;27(2):1481-1505. [ FREE Full text ] [ CrossRef ] [ Medline ] Bian Y, Yang C, Gao F, Li H, Zhou S, Li H, et al. A framework for physiological indicators of flow in VR games: construction and preliminary evaluation. Pers Ubiquit Comput. 2016;20(5):821-832. [ CrossRef ] Hossein Aqajari SA, Naeini EK, Mehrabadi MA, Labbaf S, Dutt N, Rahmani AM. pyEDA: an open-source Python toolkit for pre-processing and feature extraction of electrodermal activity. Procedia Comput Sci. 2021;184:99-106. [ CrossRef ] Scheltinga BL. Suitable metrics for upper limb movement smoothness during stroke recovery. University of Twente Student Theses. 2019. URL: http://essay.utwente.nl/79734/ [accessed 2023-08-28] Delorme A, Makeig S. EEGLAB: an open source toolbox for analysis of single-trial EEG dynamics including independent component analysis. J Neurosci Methods. 2004;134(1):9-21. [ CrossRef ] [ Medline ] Kleckner IR, Jones RM, Wilder-Smith O, Wormwood JB, Akcakaya M, Quigley KS, et al. Simple, transparent, and flexible automated quality assessment procedures for ambulatory electrodermal activity data. IEEE Trans Biomed Eng. 2018;65(7):1460-1467. [ FREE Full text ] [ CrossRef ] [ Medline ] What is a normal pulse rate? British Heart Foundation. URL: https://www.bhf.org.uk/informationsupport/heart-matters-magazine/medical/ask-the-experts/pulse-rate [accessed 2023-08-28] Verschuren O, Maltais DB, Takken T. The 220-age equation does not predict maximum heart rate in children and adolescents. Dev Med Child Neurol. 2011;53(9):861-864. [ FREE Full text ] [ CrossRef ] [ Medline ] Enewoldsen NM. Analysis of the quality of electrodermal activity and heart rate data recorded in daily life over a period of one week with an E4 wristband. University of Twente Student Theses. 2016. URL: http://essay.utwente.nl/70244/ [accessed 2023-08-28] Tabachnick B, Fidell L. Using Multivariate Statistics. 7 edition. United States. Pearson; 2018. Tukey J. Exploratory Data Analysis. 1st edition. United States. Pearson; 1977. TLX: Task Load Index VR: virtual reality Edited by A Mavragani; submitted 21.12.23; peer-reviewed by DP Broadbent, D Nath; comments to author 11.05.24; revised version received 19.06.24; accepted 15.07.24; published 23.09.24. ©Samory Houzangbe, Martin Lemay, Danielle E Levac. Originally published in JMIR Research Protocols (https://www.researchprotocols.org), 23.09.2024. This is an open-access article distributed under the terms of the Creative Commons Attribution License (https://creativecommons.org/licenses/by/4.0/), which permits unrestricted use, distribution, and reproduction in any medium, provided the original work, first published in JMIR Research Protocols, is properly cited. The complete bibliographic information, a link to the original publication on https://www.researchprotocols.org, as well as this copyright and license information must be included.
Empatica Frequently Asked Questions (FAQ)
When was Empatica founded?
Empatica was founded in 2011.
Where is Empatica's headquarters?
Empatica's headquarters is located at 1 Broadway, Cambridge.
What is Empatica's latest funding round?
Empatica's latest funding round is Series B - II.
How much did Empatica raise?
Empatica raised a total of $32.19M.
Who are the investors of Empatica?
Investors of Empatica include RA Capital Management, Sanofi Ventures, Black Opal Ventures, CDP Venture Capital, Innogest Capital and 4 more.
Who are Empatica's competitors?
Competitors of Empatica include Circadian Health, Oura, Dreem, Dozee, Biofourmis and 7 more.
What products does Empatica offer?
Empatica's products include Empatica Health Monitoring Platform and 1 more.
Who are Empatica's customers?
Customers of Empatica include Pear Therapeutics and European Union H2020.
Loading...
Compare Empatica to Competitors
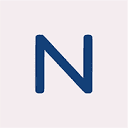
NYX serves as a neuro-technology company focused on sleep and wellness. The company offers a wearable headset designed for sleep quality using adaptive and science-backed methods. NYX primarily serves the health and wellness technology sector. It was founded in 2016 and is based in Haifa, Israel.
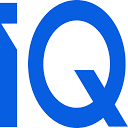
PhysIQ specializes in remote patient monitoring and data analytics within the healthcare and life sciences sectors. The company offers a platform that leverages artificial intelligence and wearable biosensors to continuously monitor patients and provide personalized medical insights, aiming to improve patient outcomes and assist in clinical trials. PhysIQ primarily serves healthcare providers and life sciences companies, offering solutions for virtual care, hospital-at-home models, and decentralized clinical research. PhysIQ was formerly known as VGBio. It was founded in 2005 and is based in Chicago, Illinois.
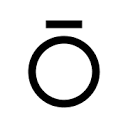
Oura is a health technology company focused on personal health and well-being through sleep and activity tracking. The company offers the Oura Ring, a wearable device that monitors various physiological signals to provide insights into sleep patterns and daily activity levels. Oura primarily serves the personal health and wellness sector, with a focus on individuals seeking to enhance their lifestyle and well-being. It was founded in 2013 and is based in Oulu, Finland.
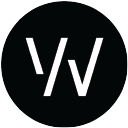
Whoop focuses on wearable technology designed to enhance human performance within the health and fitness industry. The company offers a wearable device that tracks sleep, strain, recovery, and health metrics to provide personalized insights and recommendations. Whoop primarily serves individuals seeking to improve their personal health and athletic performance. Whoop was formerly known as Bobo Analytics. It was founded in 2012 and is based in Boston, Massachusetts.
Palta develops a range of application-based products in the digital health segment. It provides insights into the health and behavioral habits of customers and helps them detect health problems through artificial intelligence (AI) systems. Its applications focus on preventative healthcare and well-being. It was formerly known as Haxus Ventures. It was founded in 2016 and is based in London, United Kingdom.
Flywallet is a fintech startup that focuses on integrating payment, mobility, and health services into a single ecosystem using proprietary wearable and IoT technologies. The company's main product, Keyble, is a wearable device that can be inserted into fashion accessories and uses biometric sensors for user authentication and health monitoring. It enables users to make payments, access online services without passwords, use public transport ticketing, manage loyalty cards, access physical locations like gyms and offices, and even unlock car doors. The company primarily serves electronic money and payment institutions, banks, insurance companies, and firms. It is based in Montopoli di Sabina, Italy.
Loading...