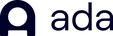
Ada
Founded Year
2016Stage
Series C | AliveTotal Raised
$190.73MValuation
$0000Last Raised
$130M | 3 yrs agoMosaic Score The Mosaic Score is an algorithm that measures the overall financial health and market potential of private companies.
+17 points in the past 30 days
About Ada
Ada is a company specializing in AI-powered customer service automation within various business sectors. The company offers a platform that enables enterprises to resolve customer inquiries efficiently across multiple channels and languages, without the need for extensive human intervention. Ada's solutions cater to industries such as ecommerce, FinTech, SaaS, and gaming. It was founded in 2016 and is based in Toronto, Ontario.
Loading...
Ada's Product Videos
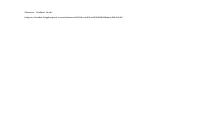
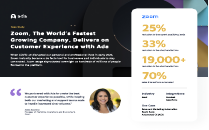
ESPs containing Ada
The ESP matrix leverages data and analyst insight to identify and rank leading companies in a given technology landscape.
The generative AI — customer support operations market offers solutions to enhance customer support experiences and streamline operations. Generative AI can automate and optimize various aspects of customer service using chatbots, virtual assistants, ticket routing, and response generation. These solutions leverage natural language processing and machine learning algorithms to understand customer …
Ada named as Challenger among 15 other companies, including Microsoft, Talkdesk, and Aisera.
Loading...
Research containing Ada
Get data-driven expert analysis from the CB Insights Intelligence Unit.
CB Insights Intelligence Analysts have mentioned Ada in 8 CB Insights research briefs, most recently on Sep 11, 2024.
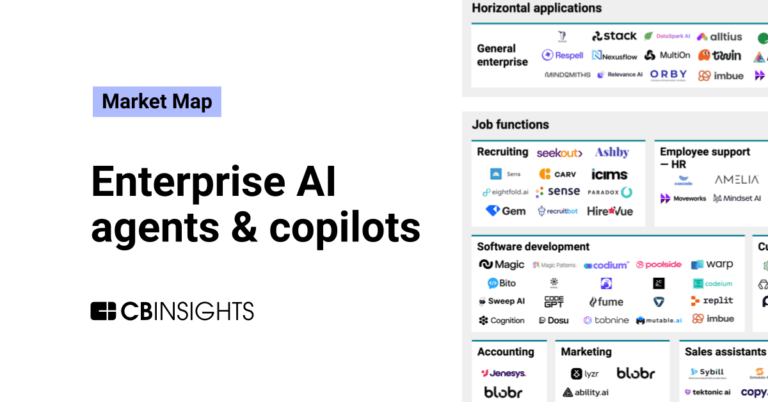
Aug 7, 2024
The enterprise AI agents & copilots market map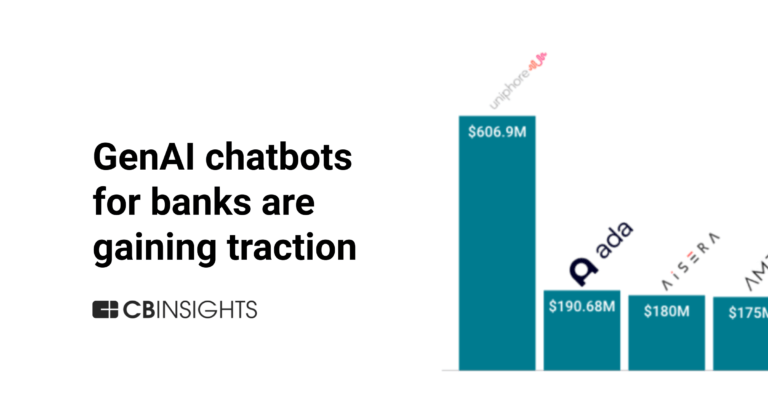
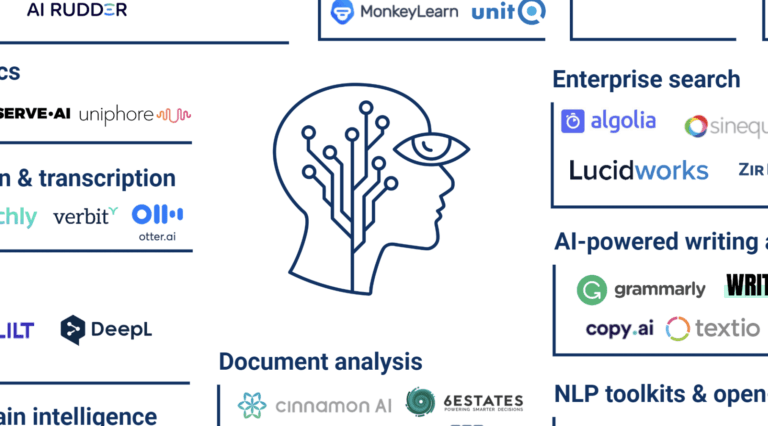
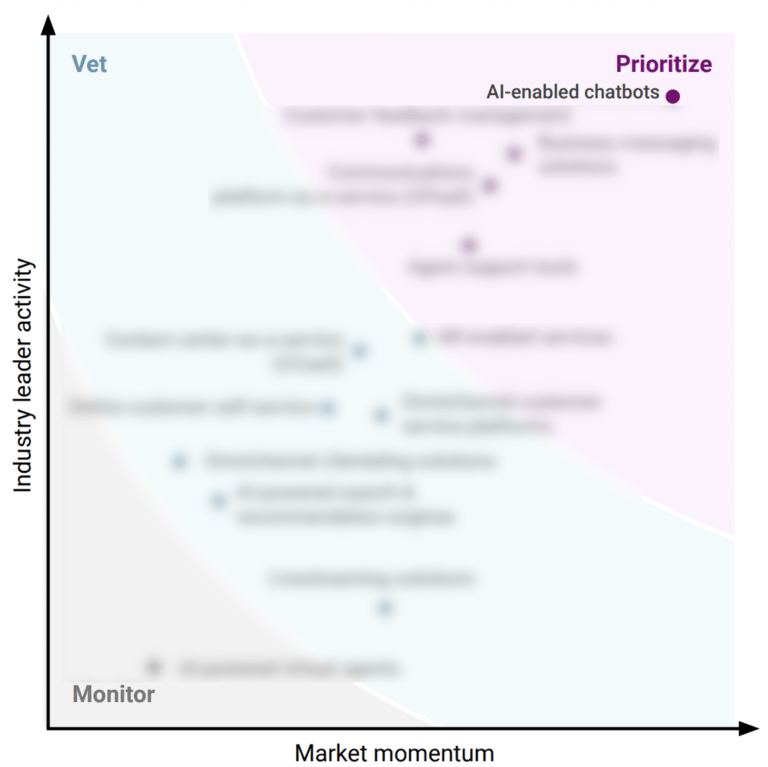
Apr 15, 2022 report
Why consumer & retail leaders are prioritizing AI-enabled chatbotsExpert Collections containing Ada
Expert Collections are analyst-curated lists that highlight the companies you need to know in the most important technology spaces.
Ada is included in 3 Expert Collections, including Unicorns- Billion Dollar Startups.
Unicorns- Billion Dollar Startups
1,244 items
Artificial Intelligence
15,089 items
Companies developing artificial intelligence solutions, including cross-industry applications, industry-specific products, and AI infrastructure solutions.
Sales & Customer Service Tech
1,091 items
Companies offering technology-driven solutions for brands and retailers to enable customer service before, during, and after in-store and online shopping.
Ada Patents
Ada has filed 7 patents.
The 3 most popular patent topics include:
- artificial intelligence
- artificial neural networks
- computational linguistics
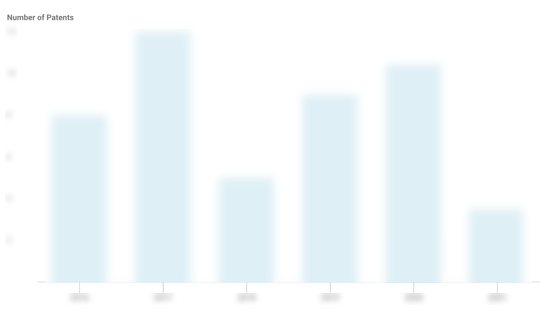
Application Date | Grant Date | Title | Related Topics | Status |
---|---|---|---|---|
6/28/2021 | 1/16/2024 | Natural language processing, Computational linguistics, Machine learning, Artificial neural networks, Artificial intelligence | Grant |
Application Date | 6/28/2021 |
---|---|
Grant Date | 1/16/2024 |
Title | |
Related Topics | Natural language processing, Computational linguistics, Machine learning, Artificial neural networks, Artificial intelligence |
Status | Grant |
Latest Ada News
Jul 23, 2024
Journal of Medical Internet Research This paper is in the following e-collection/theme issue: December 15, 2023 . Diagnostic Accuracy of a Mobile AI-Based Symptom Checker and a Web-Based Self-Referral Tool in Rheumatology: Multicenter Randomized Controlled Trial Diagnostic Accuracy of a Mobile AI-Based Symptom Checker and a Web-Based Self-Referral Tool in Rheumatology: Multicenter Randomized Controlled Trial Authors of this article: 2AGEIS, Université Grenoble Alpes, Grenoble, France 3Department of Internal Medicine 3, Friedrich-Alexander-University Erlangen-Nürnberg and Universitätsklinikum Erlangen, Erlangen, Germany 4Deutsches Zentrum für Immuntherapie (DZI), Friedrich-Alexander-University Erlangen-Nürnberg and Universitätsklinikum Erlangen, Erlangen, Germany 5Center for Health Services Research, Brandenburg Medical School Theodor Fontane, Rüdersdorf, Germany 6Faculty of Health Sciences Brandenburg, Brandenburg Medical School Theodor Fontane, Potsdam, Germany 7Verein zur Förderung der Rheumatologie e.V., Würselen, Germany 8RheumaDatenRhePort (rhadar), Planegg, Germany 10Klinik für Internistische Rheumatologie, Rhein-Maas Klinikum, Würselen, Germany 11Rheumatologische Schwerpunktpraxis, Drs. Kleinert, Rapp, Ronneberger, Schuch u. Wendler, Erlangen, Germany 12Department of Rheumatology, University Hospital Düsseldorf, Medical Faculty of Heinrich Heine University, Düsseldorf, Germany 13Institut Universitaire de France, Paris, France 14LabCom Telecom4Health, Orange Labs & Université Grenoble Alpes, CNRS, Inria, Grenoble INP-UGA, Grenoble, France 15MVZ für Rheumatologie Dr. Martin Welcker GmbH, Planegg, Germany 16Division of Rheumatology, Klinikum Nürnberg, Paracelsus Medical University, Nürnberg, Germany *these authors contributed equally Abstract Background: The diagnosis of inflammatory rheumatic diseases (IRDs) is often delayed due to unspecific symptoms and a shortage of rheumatologists. Digital diagnostic decision support systems (DDSSs) have the potential to expedite diagnosis and help patients navigate the health care system more efficiently. Objective: The aim of this study was to assess the diagnostic accuracy of a mobile artificial intelligence (AI)–based symptom checker (Ada) and a web-based self-referral tool (Rheport) regarding IRDs. Methods: A prospective, multicenter, open-label, crossover randomized controlled trial was conducted with patients newly presenting to 3 rheumatology centers. Participants were randomly assigned to complete a symptom assessment using either Ada or Rheport. The primary outcome was the correct identification of IRDs by the DDSSs, defined as the presence of any IRD in the list of suggested diagnoses by Ada or achieving a prespecified threshold score with Rheport. The gold standard was the diagnosis made by rheumatologists. Results: A total of 600 patients were included, among whom 214 (35.7%) were diagnosed with an IRD. Most frequent IRD was rheumatoid arthritis with 69 (11.5%) patients. Rheport’s disease suggestion and Ada’s top 1 (D1) and top 5 (D5) disease suggestions demonstrated overall diagnostic accuracies of 52%, 63%, and 58%, respectively, for IRDs. Rheport showed a sensitivity of 62% and a specificity of 47% for IRDs. Ada’s D1 and D5 disease suggestions showed a sensitivity of 52% and 66%, respectively, and a specificity of 68% and 54%, respectively, concerning IRDs. Ada’s diagnostic accuracy regarding individual diagnoses was heterogenous, and Ada performed considerably better in identifying rheumatoid arthritis in comparison to other diagnoses (D1: 42%; D5: 64%). The Cohen κ statistic of Rheport for agreement on any rheumatic disease diagnosis with Ada D1 was 0.15 (95% CI 0.08-0.18) and with Ada D5 was 0.08 (95% CI 0.00-0.16), indicating poor agreement for the presence of any rheumatic disease between the 2 DDSSs. Conclusions: To our knowledge, this is the largest comparative DDSS trial with actual use of DDSSs by patients. The diagnostic accuracies of both DDSSs for IRDs were not promising in this high-prevalence patient population. DDSSs may lead to a misuse of scarce health care resources. Our results underscore the need for stringent regulation and drastic improvements to ensure the safety and efficacy of DDSSs. Trial Registration: German Register of Clinical Trials DRKS00017642; https://drks.de/search/en/trial/DRKS00017642 J Med Internet Res 2024;26:e55542 Keywords Introduction Symptoms caused by inflammatory rheumatic diseases (IRDs) are often unspecific and difficult to correctly interpret for patients [ 1 ] and even for experienced rheumatologists [ 2 ]. This diagnostic complexity frequently results in significant delay [ 3 ], which can diminish treatment efficacy and lead to progressive damage [ 4 ]. To address these challenges, a variety of freely available, patient-centered diagnostic decision support systems (DDSSs) have emerged and are increasingly being used by the general public [ 5 ] and patients with IRDs [ 6 ]. These DDSSs offer disease suggestions and advice for action within a few minutes and without any health care provider contact. Rheport [ 7 ] is a web-based rheumatology referral system used in Germany to automatically triage appointments of new patients according to IRD probability [ 8 , 9 ]. To date, Rheport has been used to schedule more than 3000 appointments [ 10 ]. Ada [ 11 ], an artificial intelligence (AI)–based chatbot, is one of the most promising DDSS currently available. Multiple case-vignette studies showcased its high diagnostic accuracy [ 5 , 12 ], and more than 30 million symptom assessments have been completed in 130 countries [ 13 ]. Despite the expanding usage, little evidence is available regarding the accuracy of DDSSs in rheumatology [ 14 - 16 ]. To our knowledge, Rheport and Ada are among the most widely used DDSSs in rheumatology within Germany [ 10 , 13 ]. However, a direct comparative study between these 2 systems has yet to be conducted. Therefore, the aim of this analysis was to evaluate the diagnostic capability of Ada and Rheport in identifying IRDs. Methods Study Design and Participants The study design for this pragmatic, prospective, multicenter, crossover randomized controlled trial (German Register of Clinical Trials DRKS00017642) has been described elsewhere in detail [ 9 ]. Results are presented according to the CONSORT-EHEALTH checklist ( Multimedia Appendix 1 ) [ 17 ]. Adult patients with musculoskeletal symptoms who had been referred for the first time to 3 recruiting rheumatology outpatient clinics with a suspected diagnosis of an IRD were consecutively included in this study. Participants were instructed to enter the required data into both Ada and Rheport while waiting for their scheduled appointment with the rheumatologist. Assistance from support staff was available if needed. Patients were randomized 1:1 by computer-generated block randomization into group 1 (first Ada, then Rheport) or group 2 (first Rheport, then Ada), with each block comprising 100 patients. This crossover design was chosen to mitigate potential bias from a priming effect, where completing the first DDSS could influence responses to the second DDSS without the patient’s awareness. For instance, a priming effect was previously observed where participants who answered questions about their religiosity before reporting their alcohol consumption indicated fewer drinks on peak drinking occasions [ 18 ]. A designated project manager, uninvolved in the recruitment process, was responsible for assigning patients to the intervention arms. The statistician was kept blinded for group allocation. Assistance from the study personnel was available for DDSS completion when needed. Ethical Considerations The study was approved by the ethics committee of the medical faculty of the University of Erlangen-Nürnberg, Germany (106_19 Bc), and was conducted in compliance with the Declaration of Helsinki. All patients provided written informed consent. The DDSSs Rheport [ 7 ] comprises a static 23-item questionnaire designed to assess symptoms and generate an expert-derived weighted sum score [ 8 , 9 ]. Median completion time was 8 minutes [ 19 ]. A higher sum score correlates with an increased probability of an IRD. Rheumatologists utilizing this system can allocate slots for automatic patient scheduling. Based on the calculated IRD probability, patients are offered available appointments categorized into 4 urgency levels. Patients with scores below 1 are considered unlikely to have an IRD and do not receive an appointment. Those with a minimum score of 1 are considered likely to have an IRD and are enabled to book an appointment. The urgency levels are categorized as follows: patients with scores between 1 and 2.4 are considered intermediate, patients with scores between 2.4 and 4 are considered urgent, and patients with scores exceeding 4 are considered very urgent. Patients in the very urgent category should ideally receive appointments within 1 week [ 19 ]. Upon the acceptance of a proposed appointment by a patient, the rheumatologist is notified and provided with a structured summary report of the questionnaire. There were no changes to the Rheport algorithm during the study period. Ada [ 11 ] is a native app and certified medical product, designed to cover a broad spectrum of symptoms and diseases. Programmed as a chatbot, Ada mimics traditional history taking by initially requesting basic health information, such as sex and age, followed by current symptoms. Based on these responses, the app generates individualized follow-up questions. Ada’s diagnostic suggestions are driven by a Bayesian network that is continuously updated [ 20 ]. Upon the completion of symptom querying (median time: 7 minutes [ 19 ]), a summary report is generated, including (1) a summary of present, absent, and uncertain symptoms; (2) up to 5 disease suggestions with the corresponding probabilities, triage recommendations (eg, call an ambulance), and symptom relevance; and (3) access to basic information about the suggested diseases. Ada was regularly updated along the course of the study to ensure functionality. Outcome The primary end point of the study was concordant detection of any IRD diagnosis (including, eg, rheumatoid arthritis or systemic lupus erythematosus) by the DDSSs and the gold standard, that is, the rheumatologist’s final diagnosis, reported on the discharge summary report and adjudicated by the attending head physician of the local rheumatology department. For Rheport, the detection of an IRD by the DDSS was defined as a sum score of 1 or higher. Regarding Ada, we analyzed whether there was an IRD diagnosis and whether the correct diagnosis was listed as the top diagnosis (Ada top 1 [D1]) or was listed at all among all suggested diagnoses (Ada top 5 [D5]). Statistical Analysis Descriptive characteristics are presented as median and IQR for interval data and as absolute (n) and relative frequency (percentage) for nominal data. The minimum necessary sample size for this study was 122 in order to detect a specificity and sensitivity of at least 70% for Ada or Rheport, with a type I error of 4.4% and type II error of 19% using a 1-sample test [ 21 ] against a benchmark accuracy of 50% based on a previous evaluation of DDSSs [ 14 ]. Operating characteristics of Ada and Rheport for a diagnosis of rheumatic disease was evaluated using sensitivity, specificity, negative predictive value, positive predictive value, and overall accuracy with respective 95% CIs. This evaluation was done both separately and for the combined use of the DDSSs. The agreement between the DDSSs was evaluated using the Cohen κ statistic, with values ≤0 indicating no agreement, 0.01-0.20 indicating none to slight agreement, 0.21-0.40 indicating fair agreement, 0.41-0.60 indicating moderate agreement, 0.61-0.80 indicating substantial agreement, and 0.81-1.00 indicating almost perfect agreement [ 22 ]. We evaluated the cumulative proportion of correct diagnoses using Ada with exact CIs. We used a binomial regression with a log-link function to calculate the risk ratio for correct identification of any IRD by Rheport in comparison to Ada when the respective DDSS was used first and when it was used after the crossover. We preferred this method over logistic regression since the interpretation of risk ratios is more intuitive than that for odds ratios with high-prevalence binary outcomes. All analyses were conducted using the open-source R software (version 4.1.0; R Foundation for Statistical Computing) running under RStudio (version 1.4.1103; RStudio). Results Participants A total of 755 consecutive patients were approached between September 2019 and April 2021, of whom 654 (87%) agreed to participate and 600 (79.4%) were included in the analysis presented ( Figure 1 ). The participation exceeded the minimal sample size calculation, since patients were very eager to participate and considered the study a welcome distraction during the waiting time for their appointment. Overall, 35.7% (214/600) of the patients were diagnosed with an IRD based on physicians’ judgment. The demographic characteristics of the patients are displayed in Table 1 , and the physicians’ final diagnoses are presented in Table 2 . Figure 1. Patient flow diagram. Table 1. Patient demographic characteristics according to the physicians’ final diagnosis, reported on the discharge summary report. Demographics Diagnostic Accuracy of Ada and Rheport Rheport showed an overall sensitivity of 62% and a specificity of 47% for IRDs ( Figure 2 ). Ada’s D1 and D5 disease suggestions showed a sensitivity of 52% and 66%, respectively, and a specificity of 68% and 54%, respectively, concerning IRDs ( Table 3 ). The odds ratio for Rheport correctly suggesting a rheumatic disease diagnosis in comparison to Ada D5 as the first used DDSS was 0.89 (95% CI 0.83-0.97). When the initial DDSS was Ada, the accuracy of Ada D5 was 61% (95% CI 55%-66%) and the accuracy of Rheport was 53% (95% CI 47%-59%), whereas after the crossover, this odds ratio was 0.98 (95% CI 0.91-1.06) with corresponding accuracies of Ada D5 at 56% (95% CI 50%-61%) and of Rheport at 52% (95% CI 46%-58%). Ada’s diagnostic accuracy regarding individual diagnoses was heterogenous. Ada suggested the correct diagnosis of as top suggestion (Ada D1) in 42% (29/69) of patients with rheumatoid arthritis, and the correct diagnosis was suggested overall (Ada D5) in 64% (44/69); moreover, the first suggestion of Ada (Ada D1) was correct in 22% (14/65) of patients with spondyloarthritis (including axial spondyloarthritis, peripheral spondyloarthritis, and psoriatic arthritis), and the correct diagnosis was suggested overall (Ada D5) in 38% (25/65). The findings suggest that Ada performed considerably better in identifying rheumatoid arthritis in comparison to other diagnoses (see Figure 3 ). Figure 2. Diagnostic properties of Ada and Rheport regarding IRDs according to the order of usage. Ada D1: Ada's top diagnosis; Ada D5: Ada's top 5 suggestions; IRD: inflammatory rheumatic disease; DDSS: diagnostic decision support system. Table 3. Sensitivity, specificity, PPVa, NPVb, and overall accuracy of Ada and Rheport for the diagnosis of inflammatory rheumatic diseases including 95% CIs. Characteristic
Ada Frequently Asked Questions (FAQ)
When was Ada founded?
Ada was founded in 2016.
Where is Ada's headquarters?
Ada's headquarters is located at 371 Front Street West, Toronto.
What is Ada's latest funding round?
Ada's latest funding round is Series C.
How much did Ada raise?
Ada raised a total of $190.73M.
Who are the investors of Ada?
Investors of Ada include Version One Ventures, Bessemer Venture Partners, FirstMark Capital, Burst Capital, Accel and 10 more.
Who are Ada's competitors?
Competitors of Ada include Botco.ai, Cognigy, PolyAI, Mavenoid, Ultimate and 7 more.
Loading...
Compare Ada to Competitors
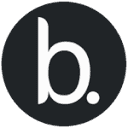
Inbenta specializes in conversational AI and automation within the customer service sector. The company offers a suite of products including AI-enabled chatbots, search tools, and knowledge management systems designed to enhance customer experience and streamline service operations. Inbenta's conversational AI platform is utilized across various industries to automate customer interactions, provide self-service options, and improve overall customer satisfaction. It was founded in 2005 and is based in Sunnyvale, California.

Pypestream specializes in AI-powered customer experience automation within various business sectors, including insurance, telecommunications, retail, and more. The company offers an omnichannel platform that leverages conversational AI to provide personalized, efficient customer service and support through a library of microapps. Pypestream's solutions are primarily utilized by industries such as insurance, telecommunications, retail, quick service restaurants, travel, hospitality, and real estate. It was founded in 2015 and is based in New York, New York.
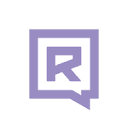
Rasa focuses on providing enterprise-grade for business sectors. The company offers a suite of products that enable personalized and scalable customer interactions through text- and voice-based AI. Rasa's solutions cater to industries such as financial services, healthcare, telecom, and travel and transport. Rasa was formerly known as LastMile Technologies. It was founded in 2016 and is based in San Francisco, California.

Cognigy specializes in conversational artificial intelligence (AI) platforms and customer service automation within the technology sector. It offers AI-powered virtual agents that facilitate customer service interactions through voice and digital chat and support contact center operations. Its solutions are utilized across various industries, including airlines, automotive, financial services, healthcare, insurance, retail, telecommunications, and utilities. The company was founded in 2016 and is based in Dusseldorf, Germany.
ICM Hub specializes in conversational AI within the airline industry, offering intelligent bot solutions to streamline customer interactions. The company provides automated chat support that covers various stages of the airline customer experience, including pre-flight, in-flight, and post-flight services, aiming to enhance customer engagement and operational efficiency. ICM Hub's solutions are primarily tailored for the airline and travel sectors, focusing on improving customer satisfaction and increasing ancillary sales. It was founded in 2016 and is based in New York, New York.
Intercom specializes in customer support technology within the tech industry. It offers a range of products, including a help desk, an AI chatbot, proactive support tools, and a platform for delivering personalized support at scale. The company primarily serves sectors such as eCommerce, education, healthcare, and financial services. It was founded in 2011 and is based in San Francisco, California.
Loading...