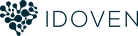
Idoven
Founded Year
2018Stage
Incubator/Accelerator - V | AliveTotal Raised
$23.11MMosaic Score The Mosaic Score is an algorithm that measures the overall financial health and market potential of private companies.
-154 points in the past 30 days
About Idoven
Idoven is a health technology company focused on detection and medicine in the cardiovascular domain. The company offers an artificial intelligence (AI)-powered platform that improves the speed, consistency, and accuracy of electrocardiogram (ECG) interpretation, and works with existing ECG devices to support clinician decision-making and disease management. It was founded in 2018 and is based in Madrid, Spain.
Loading...
Idoven's Product Videos
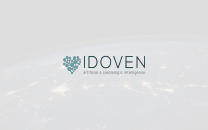
ESPs containing Idoven
The ESP matrix leverages data and analyst insight to identify and rank leading companies in a given technology landscape.
The radiology AI — electrocardiogram (ECG) diagnostics market focuses on the development and adoption of AI-powered solutions designed to assist in the interpretation and analysis of electrocardiogram (ECG) data. ECG is a critical diagnostic tool for assessing the electrical activity of the heart, and AI applications in this market aim to improve the accuracy and efficiency of diagnosing cardiac c…
Idoven named as Outperformer among 11 other companies, including Tempus, Viz.ai, and AliveCor.
Idoven's Products & Differentiators
WillemTM
AI Platform
Loading...
Research containing Idoven
Get data-driven expert analysis from the CB Insights Intelligence Unit.
CB Insights Intelligence Analysts have mentioned Idoven in 2 CB Insights research briefs, most recently on Dec 5, 2023.
Expert Collections containing Idoven
Expert Collections are analyst-curated lists that highlight the companies you need to know in the most important technology spaces.
Idoven is included in 4 Expert Collections, including Artificial Intelligence.
Artificial Intelligence
14,767 items
Companies developing artificial intelligence solutions, including cross-industry applications, industry-specific products, and AI infrastructure solutions.
Digital Health
11,072 items
The digital health collection includes vendors developing software, platforms, sensor & robotic hardware, health data infrastructure, and tech-enabled services in healthcare. The list excludes pureplay pharma/biopharma, sequencing instruments, gene editing, and assistive tech.
Telehealth
2,918 items
Companies developing, offering, or using electronic and telecommunication technologies to facilitate the delivery of health & wellness services from a distance. *Columns updated as regularly as possible; priority given to companies with the most and/or most recent funding.
Digital Health 50
200 items
2022's cohort of the most promising digital health startups transforming the healthcare industry. Winners were chosen based on several factors, including data submitted by the companies, proprietary Mosaic scores, company business models and momentum in the market.
Latest Idoven News
Sep 18, 2024
Machine learning in healthcare: Uses, benefits and pioneers in the field 18th September 2024 Artificial Intelligence (AI) and machine learning (ML) are so much more than Chat GPT, image creators, and other ways of filling the internet with even more content. In a world with data is everything, machine learning can help us analyse and understand this information more effectively. It can help us make breakthrough discoveries and create innovative solutions to improve people’s lives. This is particularly true in the healthcare sector. In healthcare, machine learning has the potential to transform patient outcomes, enhance the quality of care, streamline healthcare delivery, and, more importantly, make healthcare more accessible and cost-effective. In recent years, predictive healthcare models have been developed that can accurately identify trends, patterns, and subtle abnormalities that may escape even the most experienced professional, and, as such, applications of machine learning are growing rapidly, from early diagnosis of diseases to predictive analytics and personalised medicine. How is machine learning used in medicine? From diagnostics to personalised medicine, machine learning is helping research institutions, companies, and start-up innovate and create transformative solutions across the healthcare sector, including: Research In a lab environment, machine learning can help researchers understand how a disease really works and improve hypothesis testing. Through analysis of advanced data sets, algorithms can simulate disease progression which gives researchers insights into how conditions develop and how they respond to treatment. This is especially valuable for diseases like cancer, where being able to predict how a patient is likely to respond to specific drugs can lead to more personalised treatment strategies. Additionally, by using machine learning researchers can develop a hypothesis model, then adjust, refine and replicate the process iteratively based on the evolving data. This process can improve prediction accuracy and improve collaborative efforts between clinicians and data scientists thereby accelerating the development of innovation solutions. Disease Diagnosis and Prediction Traditionally, disease diagnosis relied on the experience of the healthcare professional, and to some extent their intuition, along with available tests. But because machine learning can analyse huge amounts of data faster and identify subtle patterns and correlations far more accurately than humans, it is quickly transforming the field of diagnostics. For example, in radiology, machine learning models are trained to examine medical images such as X-rays, MRIs, and CT scans, detecting anomalies like tumours or lesions with remarkable precision. These models are particularly useful for early-stage diagnosis for diseases such as cancer or cardiovascular conditions where early detection is critical for successful treatment outcomes and patient survival. Beyond diagnostics, by analysing individual patient data including lifestyle factors, genetic predispositions, and previous medical conditions, machine learning is being used in predicting the probability of a patient developing a certain disease, its onset and progression. This allows healthcare providers time to provide personalised care and implement preventative measures and monitoring strategies before a disease becomes symptomatic, which improves patient outcomes. Personalised medicine and treatment plans Personalisation is one of the most promising developments in modern medicine. Instead of patients following a one-size-fits-all approach, machine learning can analyse individual patient data to enable healthcare providers to predict which treatment option is likely to be the most effective, minimising the risk of an adverse reaction. This approach is most valuable in fields like oncology, where treatment effectiveness varies enormously between patients. And because machine learning models are continuously learning from new data, this means personalised treatment plans are dynamic and adaptable. So if a treatment is or isn’t working, the model can suggest adjustments to dosage or medication, meaning it’s continuously being optimised to suit the patient’s evolving condition and ensuring the treatment remains effective, which is especially useful in managing chronic diseases such as hypertension or diabetes. Additionally, machine learning-driven personalised treatment plans can predict when a patient is most likely to stray from their treatment plan and put into motion interventions such as reminders or follow-up appointments to help patients stick to the plan. Drug discovery and development Machine learning is helping researchers with drug development by helping to accurately identify potential new drugs, and predict their safety and effectiveness through: Analysing complex biological data such as genetic information, protein structures, and molecular interactions, and predicting how different compounds will interact with various target proteins and enzymes. Modelling and predicting how changes to the chemical structure of the drug will affect its performance to ensure that it is safe for patients. This also leads to drugs that are more likely to succeed in the clinical trial phase. Analysing past clinical trial data to predict how the new drug might work along with any potential risks. This analysis can also enhance the trial’s design so that it run more efficiently and effectively by identifying suitable patient populations, appropriate dosing strategies, and potential side effects. When it comes to existing drug data, machine learning analysis can identify repurposing opportunities and predict potential new therapeutic uses for known compounds. Predictive analytics for patient outcomes Using machine learning algorithms to analyse electronic health records (EHRs) and other data sources such as genetic information and wearable devices, predictive analytics can model the chances of disease progression to risks of complications and hospital admission/readmission, which enables healthcare providers to proactively address potential health issues before they become critical, ensuring that all interventions are timely, targeted and effective. The most common applications of predictive analytics in healthcare are: Chronic disease management: Models flag when a patient’s condition shows early signs of deterioration based on their historical data and current health indicators, prompting healthcare providers to adjust medications, recommend lifestyle changes, or increase monitoring. Treatment response (mainly in oncology): Using data from patients with similar profiles, machine learning algorithms can predict which chemotherapy regimens or targeted therapies are the most likely to be effective. This reduces the trial-and-error approach that can delay effective care and cause unnecessary side effects. Post-surgical care: Analysis of pre-existing conditions, surgery complexity, and recovery data models can identify patients likely to have longer recovery times and those at risk of post-surgery complications, such as infections and blood clots. This enables healthcare providers to take preventative action to improve recovery outcomes and reduce readmission risks. Medication management: By predicting how patients with specific genetic profiles will respond to certain medication, healthcare providers can prescribe those drugs that will be most effective and least likely to cause side effects. Preventive care: For patients at high risk of developing chronic conditions based on individual factors such as family history, lifestyle choices and early warning signs, healthcare providers can implement preventive measures. This leads to better long-term health outcomes and can reduce the problem chronic diseases have on patients and the whole healthcare system. Improving operational efficiency Beyond direct patient care, machine learning can help improve operational efficiency and reduce costs across the healthcare system without compromising patient care: Optimising resource allocation: Healthcare environments, especially hospitals, often struggle with the unpredictable nature of patient admissions, leading to overstaffing or understaffing, which can either waste resources or compromise patient care. Machine learning models can analyse historical data, such as patient admission rates, seasonal trends, and local population health patterns, to predict future demand for services. Administrative tasks: Appointment scheduling, billing, and patient management, are all tasks that are time-consuming and can be prone to errors when done manually. Automating these tasks with machine learning algorithms creates a win-win situation for everyone, by reducing appointment wait time, speeding up payment cycles, and reducing time spent of paperwork. Supply chain management: By predicting demand for supplies, optimising inventory levels, and reducing waste, machine learning can help healthcare facilities reduce the costs as well as identifying the best sources for high-quality, cost-effective supplies. Patient flow management: Efficient patient flow management is essential for reducing bottlenecks and ensuring that patients receive timely care. Machine learning can predict and manage patient flow by analysing data such as patient demographics, admission patterns, and treatment durations. This can help reduce bottlenecks, whether this is managing bed availability, optimising surgery scheduling, or triaging patients in Accident and Emergency by analysing symptoms and medical history to prioritise those most in need. Benefits of machine learning in healthcare Earlier diagnosis Machine learning models have been built to accurately identify early signs of disease, leading to earlier intervention, which is crucial for treatment success and better patient outcomes. Drug development Machine learning is helping to optimise various stages of the process, accelerating the drug discovery and development process. Reducing healthcare costs Improving operational efficiency, reducing errors, and enhancing patient outcomes all helps to reduce healthcare costs while maintaining, or even improving quality of patient care. Enhancing data privacy and security The protection of sensitive patient data is a major concern as healthcare becomes digitised. Machine learning models anonymise patient data which ensures compliance with data protection regulations like GDPR, while maintaining the data’s value for research and analysis purposes. Additionally, advanced machine learning algorithms are used to alert healthcare organisations, in real time, of potential breaches or unauthorised access. Improving Patient Care Personalised medicine ensures that each patient receives the treatment that is right for them, improving its effectiveness, reducing side effects, and improving patient experience, but this is only possible with the help of machine learning. By monitoring patient health in real-time, machine learning models can support long-term health outcomes by providing continuous, data-driven care to help with chronic disease management and the early detection of potential health issues. AI vs machine learning: How do they differ? The terms ‘Artificial Intelligence’ (AI) and ‘Machine Learning’ are often used interchangeably, however, in reality, they actually represent different concepts. Artificial intelligence: AI uses a wide range of technologies, from simple rule-based systems to advanced neural networks to create intelligent systems that are capable of performing tasks that would typically require human intelligence, such as reasoning, problem-solving, and understanding natural language. Machine Learning: This is a subset of AI that provides the tools and techniques needed to create those intelligent systems, and focuses on the development of algorithms that allow computers to learn from data and improve their performance over time without being explicitly programmed. Understanding the distinction between AI and machine learning is crucial for leveraging their potential. For example, in healthcare, AI might be used to design complex decision-making systems that assist in diagnostics, while machine learning is specifically employed to analyse patient data and predict outcomes, helping to tailor treatment plans to individual patients. Pioneering uses of machine learning in medicine by EIT Health-supported start-ups BiomeDX Biome Diagnostics GmbH (BiomeDx®) is an Austrian-based MedTech company working to advance precision medicine by pioneering microbiome-based technologies that transform cancer care. The company is strategically positioned at the intersection of state-of-the-art DNA sequencing and advanced machine learning algorithms, to develop first-in-class technologies for routine clinical practice. Identifying the multitude of pathways in which the symbiotic microbiome interacts with the human body is a complex undertaking. But one which holds the key for game-changing diagnostic and pharmaceutical applications that will be a quantum leap forward for doctors and patients. How BiomeDX uses machine learning BiomeDX aims to use machine learning to understand and link microbial functions in the context of complex biological metadata, including human diversity such as genetics, health status, medication, nutrition. This can help doctors understand if certain cancer patients would benefit from checkpoint inhibitor-based cancer immunotherapy. Their advanced analysis platform combines a proprietary knowledge database of bacteria-bacteria associations and thousands of microbiome profiles with advanced bioinformatics, statistical models and machine learning algorithms. This unique and medically-compliant technology enables BiomeDx and their partners to learn how the intestinal microbiome affects a patient’s health and influences diseases. How EIT Health has supported BiomeDX We have supported BiomeDx through EIT Health Catapult , a unique competition and training programme that showcases life sciences and health tech start-ups to leading experts and investors across Europe. The programme awards the best business concepts, fast-tracking start-ups to become part of the EIT Health Community of world-leading companies. BiomeDx achieved 2nd place in the 2023 EIT Health Catapult programme’s MedTech category and won the esteemed “Alex Casta Audience Award” which gave them the unique opportunity to have their logo displayed on the NASDAQ Tower in Times Square in New York, for millions to see. Milestones BiomeDx is globally the first company in the area of microbiome that holds both ISO certifications ISO 9001 (quality management systems) and ISO 13485 (medical devices), and currently has four products on the market: myBioma®: Explorative microbiome analysis based on NGS and AI (for the general public) including supplements BiomeOne®: Cancer immunotherapy response detection BiomeCRC®: Early colorectal cancer diagnostic screening test BiomeFMT: Stool donor identification for non-responders Optellum Lung diseases, which cause 15% of all deaths in European Union countries, are frequently chronic and place a large burden on healthcare systems. [1] Optellum is an Oxford-based lung health technology company using the power of artificial intelligence to revolutionise early diagnosis and treatment for lung disease patients. Their aim is to enable every clinician, in every hospital, to make the right decisions and give their patients the best chance to fight back. How Optellum uses machine learning Optellum’s AI-based lung cancer decision support software, known as the Virtual Nodule Clinic, calculates a clinically validated Lung Cancer Prediction (LCP) score using imaging AI, enabling physicians to accurately identify and assess the probability of malignancy in lung nodules. Using AI’s capabilities, Optellum is empowering healthcare professionals to make informed decisions early in the diagnostic process, ultimately leading to more effective treatments and improved patient outcomes. How EIT Health has supported Optellum In 2016, Optellum took part in EIT Health Catapult and another of our accelerator programmes, which set the stage for their growth journey. In 2017 EIT Health’s LUCINDA innovation project brought together experts in lung cancer, machine learning, and MedTech product development, and this collaboration led to the launch of Optellum’s advanced AI technology in 2021. Milestones In September 2022, Optellum secured $14 million in a Series A funding round, bringing their total equity and innovation grant funding to approximately $30 million since their inception. Corify Care Cardiac arrhythmias, such as atrial fibrillation, affect millions of people worldwide, leading to significant health risks, including stroke and heart failure. It is estimated that 17.9 million people will suffer with atrial fibrillation, the most common type of arrhythmia, in Europe by 2060. [2] Traditional methods of cardiac mapping are often invasive, time-consuming, and uncomfortable for patients. Corify Care was set up to improve the diagnosis and treatment of cardiac arrhythmia. The ACORYS MAPPING SYSTEM provides a non-invasive, rapid, and accurate method for mapping the heart’s electrical activity, eliminating the need for CT or MRI scans. By utilising body surface potential measurements and a 3D torso model, ACORYS generates highly detailed electro-anatomical maps of the heart that enables clinicians to observe the heart’s electrical activity in real-time, enhancing their ability to diagnose and treat cardiac arrhythmias with unparalleled precision, speed, and safety. How Corify Care uses machine learning ACORYS® merges advanced hardware with cutting-edge imaging and signal processing technologies to process the heart’s signal as a whole, in parallel, and in real time. This integration offers clinicians detailed electro-anatomical maps of the heart’s surface, showcasing its electrical activity through a wholly non-invasive approach. ACORYS® can generate maps in under 10 minutes without relying on other imaging systems or endocardial catheters. Moreover, it’s engineered to work synergistically with structural information like fibrosis maps from LGE-MRI or endocardial recordings via catheters, achieving a truly global mapping of the heart for the first time. How EIT Health has supported Corify Care Corify Care has participated in two EIT Health accelerator programmes aimed at helping start-ups develop their potential. In 2020, EIT Health recognised Corify Care’s technology as the most innovative in Europe. Following this recognition, in 2021 Corify Care made a significant leap forward with a private investment round of €2.3 million. Since 2022, EIT Health has continued to be an integral part of Corify Care’s journey, funding the EIT Health innovation project SAVE-COR with over €2.5 million. Milestones In July 2024 Corify Care achieved CE Mark Certification which was made possible by evidence gathered in several clinical studies conducted as part of the SAVE-COR project in referral hospitals in Spain and Portugal. These studies, involving over 1,000 patients, demonstrated the critical need for this innovative technology. Idoven Spanish-based Idoven , a health technology company advancing early detection and precision medicine for cardiovascular diseases, was founded in 2018 after almost a decade of basic and translational research in cardiology and arrhythmias. They have developed the world’s first cardiology-as-a-service platform powered by artificial intelligence that augments a clinician’s ability to identify, triage and diagnose patients at scale. Cardiovascular diseases (CVDs) are the leading cause of death globally, with approximately 18 million yearly fatalities from CVDs, representing 32% of all global deaths, according to WHO. [3] Each year CVD causes 4 million deaths in Europe, accounting for 45% of all deaths. [4] CVDs are also the single largest cost driver in the global healthcare system. How Idoven uses machine learning Idoven’s artificial intelligence platform, Willem, certified as an EU MDR Medical Device Class IIa, delivers substantial improvements to the speed, consistency and accuracy of electrocardiogram (ECG) interpretation. Its powerful, proprietary AI algorithms, which work with existing ECG devices, are also being applied to develop disease biomarkers towards patient identification, risk stratification and prognosis, as well as drug cardiac safety monitoring. Idoven partners with leading medical device and pharmaceutical companies to develop AI-based innovations to establish a new paradigm in cardiovascular healthcare. How EIT Health has supported Idoven Idoven has participated in several EIT Health accelerator programmes, which have provided them with mentorship and funding opportunities to accelerate their development, given them access to living labs to help develop market-ready products, and guided them on international market expansion. Idoven have also been supported through EIT Health’s FAITHFUL and ASSIST innovation projects, where they played a leading role in the project consortium that received our funding. Milestones Willem, Idoven’s ECG Analysis Platform has achieved CE Mark certification as a Class IIa Medical Device under Regulation (EU) 2017/745, allowing qualified healthcare professionals to assess arrhythmias using ECG data from a variety of devices, including standard 12-lead diagnostic ECGs, ambulatory devices like Holter monitors and patches, and Insertable Cardiac Monitors (ICMs). Tucuvi Tucuvi is a MedTech company that augments healthcare professionals’ capabilities, allowing them to focus their time and skills where they are most needed. Healthcare systems leverage Tucuvi’s safe and clinically validated voice AI clinical assistant to perform autonomous phone consultations, eliminating unnecessary follow-up visits and streamlining waiting lists. Their solution, Tucuvi Health Manager, is the only patient management platform with a CE-marked product for AI clinical phone conversations for +30 different pathologies and care processes. Tucuvi is augmenting healthcare professional capacities, freeing up time to use their skills where they are needed most. How Tucuvi uses machine learning LOLA is Tucuvi’s AI virtual clinical assistant that makes calls to patients. Based on Conversational AI, patients can talk to LOLA just like they would with a real healthcare professional. As an autonomous and empathetic AI, LOLA works across more than 30 different clinical pathways, conducting autonomous clinical phone conversations and looking after more than 300,000 patients. After each call, LOLA collects all the information from the conversation and using advanced algorithms this information is transferred onto a clinical dashboard (Tucuvi Dashboard) or EHR in a structured and prioritised way, allowing clinical teams to intervene more efficiently and effectively. How EIT Health has supported Tucuvi Tucuvi has participated in several EIT Health accelerator programmes where they have been supported to implement their solution by our world-class partners, including key players in the pharmaceutical industry. They have also received business support and coaching on international market expansion from our network. MJN-Neuro Epilepsy affects around 1% of the total world population and approximately 30% of people who have epilepsy are drug-resistant. [5] MJN-neuro develop technological solutions to improve the quality of life of people affected by neurological diseases, and have developed the mjn-SERAS medical device, which is like a hearing aid, to predict epileptic seizures with high reliability thanks to an Artificial Intelligence algorithm. Between 1 and 3 minutes before the seizures, an alarm alerts patients via their mobile phone. During this time, patients can reach a safe place or position to avoid accidents and injuries, helping them to become independent and improving their quality of life. How MJN-Neuro uses machine learning Powered by artificial intelligence, the device uses machine learning to personalise the algorithm to the user’s own brain activity patterns, enhancing its ability to predict future seizures. How EIT Health has supported MJN-Neuro MJN-Neuro has participated in several EIT Health accelerator programmes which have helped them to grow, including the EIT-EIC Fast Track in 2023.
Idoven Frequently Asked Questions (FAQ)
When was Idoven founded?
Idoven was founded in 2018.
Where is Idoven's headquarters?
Idoven's headquarters is located at Calle Francisco Campos, 22 - PLT BJ, Madrid.
What is Idoven's latest funding round?
Idoven's latest funding round is Incubator/Accelerator - V.
How much did Idoven raise?
Idoven raised a total of $23.11M.
Who are the investors of Idoven?
Investors of Idoven include Gobe Ventures, Insight Partners, Northzone, Salica Investments, Luis Sanz and 22 more.
Who are Idoven's competitors?
Competitors of Idoven include Anumana and 2 more.
What products does Idoven offer?
Idoven's products include WillemTM.
Who are Idoven's customers?
Customers of Idoven include Astrazeneca and FIFPRO.
Loading...
Compare Idoven to Competitors
CardioAI is focused on providing deep learning technology to real-life products for natural language processing and healthcare. It offers productivity tools for clinical diagnosis, remote patient monitoring, and off-the-shelf software for digital health applications and devices. It was founded in 2015 and is based in Riga, Latvia.

Cardiomatics is a company that focuses on the application of artificial intelligence to cardiac diagnostics and treatment, operating within the healthcare technology sector. The company offers a cloud-based AI technology that analyzes electrocardiogram (ECG) data, providing fast and accurate ECG analysis for healthcare providers. This technology is primarily used in the healthcare industry, particularly by cardiologists and other medical professionals dealing with cardiac health. It is based in Krakow, Poland.
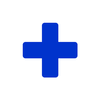
Powerful Medical specializes in artificial intelligence-driven solutions for the healthcare sector. The company offers a platform that provides interpretation of 12-lead electrocardiogram (ECG) to assist in the detection and treatment of cardiac conditions. It primarily serves the healthcare sector. The company was founded in 2017 and is based in Bratislava, Slovakia.
Spacelabs Healthcare is a company that focuses on healthcare technology, specifically in the areas of patient monitoring and diagnostic cardiology. The company offers a range of products and services including patient monitoring and connectivity solutions, cardiology and remote monitoring tools, and data management systems. These offerings are primarily used in the healthcare industry. It was founded in 1958 and is based in Snoqualmie, Washington.
Scit provides a software platform that monitors a user's health information.
XOresearch is a company that focuses on providing deep learning technology to real-life products for natural language processing and healthcare.
Loading...